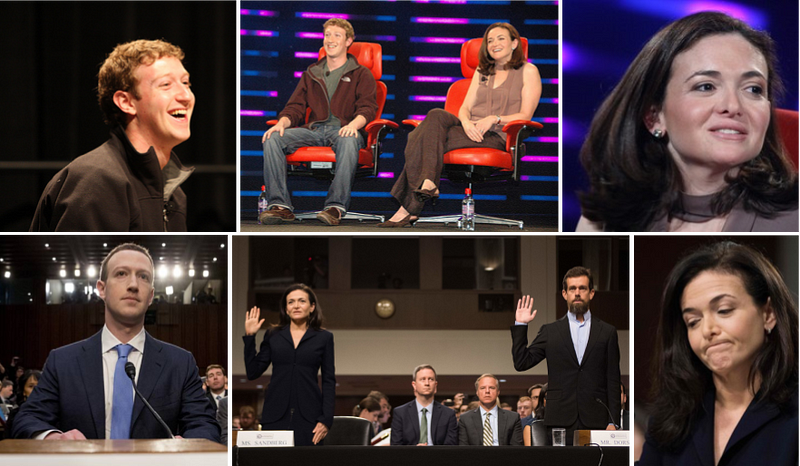
The anguish of unintended consequences
We celebrate the promise of artificial intelligence as we suffer the unintended consequences of social media. These states are linked across time.
In the formative days, we spoke of social media with the same radiant enthusiasm that now shines on artificial intelligence. With youthful optimism, we just needed to bring everyone together. The focus was overwhelmingly on the technology, on the new media, not the old social order. If you didn’t get it, you were probably too old, or too dumb, or both.
You see, it was obvious: Social media would inevitably connect and transform the world. The broader implications were to be discovered, not considered.
We now know all too well that social media reflects both the best andworst of our social instincts. Rather than correcting, social media amplify these tendencies. They entrench perspectives to such a degree that serious people consider social media a weapon of war and worry that even democracy itself might be an unintended casualty. Such is the anguish of unintended consequences.
To be fair, could anyone really imagine the consequences? As Kara Swisher recalls the history of Facebook, most didn’t really try. It wasn’t just in the founders’ naivety or willful blindness. It wasn’t thought a coherent position. Facebook was benign. Facebook was a utility. Swisher recalls, “Facebook was ‘probably too focused on just the positives and not focused enough on some of the negatives.’”
“I don’t think it is acceptable to get the same things wrong over and over again.” — Mark Zuckerberg
More damning, there was arrogance, the sense that only the architects of social media truly understood their tools. Progress could only be achieved this way, left unimpeded. The motto was move fast and break things. Mark Zuckerberg said, “In running a company, if you want to be innovative and advance things forward, I think you have to be willing to get some things wrong. But I don’t think it is acceptable to get the same things wrong over and over again.”
Yet here we are again, on the precipice of an even more profound technological change powered by AI. Are we repeating the same mistakes? Rarely a day goes by without another hype piece on the future of AI, ironically interspersed with another revelation of social media malfeasance and decay. And again, a vocal minority is expressing the same unbridled optimism, the same sense of inevitability, and yes, the same arrogance that they alone understand their tools.
Which of course is true: Only experts in AI understand AI. But unintended consequences don’t yield to experts, any more than storms yield to meteorologists. Unintended consequences envelope a much broader range of factors, which in turn demand a much more inclusive conversation.
The stakes will be even higher next time. Perhaps the most consequential of consequences lie at the intersection of AI and healthcare. Healthcare, not coincidentally, is the area most ridiculed for their slow adoption of AI. And like our social nature, medicine embodies the same indeterminable mix of good and bad that makes it particularly vulnerable to unintended consequences.
In a recent New York Times roundtable discussion on the implications of technology and medicine, Regina Barzilay expressed a sentiment that captures the moral quandary. It casts AI as a moral imperative, and an obvious one at that. “For me as a computer scientist working in artificial intelligence, it seemed obvious to train a machine to make these kinds of predictions.” Medicine has problems that AI mustaddress. “As an A.I. researcher, I was stunned to see all these opportunities to help patients squandered. From a patient’s perspective, it felt cruel.”
As Barzilay highlights, inaction is a moral choice, and forgoing opportunities to conscientiously apply AI to healthcare problems is a moral failing. But when this sentiment travels with claims to obviousness, I get nervous.
Technologists understandably focus on the containment strategy. Barzilay continued, “We’re talking about well-understood technology commercially deployed in other industries, not brand-new research.” But unfortunately, the implications aren’t neatly confined to the solutions. Recall what we’ve learned from social media and the momentous impact of 140-characters, appropriately networked. Within healthcare, among the most dire circumstances, the most complex environments, what will prevent a similar outbreak of unintended consequences?
Unfortunately, when we reason about narrowly focused solutions, vague concerns about unintended consequences feel like fear-mongering. If it can be proved through controlled experiments that AI systems outperform humans, what could possibly be the downside? This is exactly the point: Unintended consequences are discovered once these systems are actually deployed in the real world.
Consider whether improvements in the sensitivity of the diagnostics might outpace improvements in the interventions. Improved diagnostics could lead to more diagnoses, but also to more treatments of questionable value. Automation might move diagnostics from specialists to generalists, removing the checks and balances of human experts. It may free attention and resources for other problems, but also impact the vigilance, tolerance of risk, and perceptions of responsibility in clinicians. Note how these effects quickly radiate beyond the initial intentions of the diagnostic system. And this is just a shortlist of effects that can be anticipated, even if they are generally unknown outside the community of medical experts.
There is undoubtedly much greater awareness of the risks this time around. Tom Simonite called 2018 the year tech put limits on AI. It’s a chronicle of progress. But there’s a world of difference between reactive and proactive policies. Presently, we react. We have data governance policies because we’ve suffered data breaches. We demand greater transparency because secrecy is the corporate norm. If we’re going to weather unintended consequences, we need to shift to a proactive stance.
To appreciate the difference, the computer scientist Thomas G. Dietterich offers a model for proactive AI governance in the concept of “High Reliability Organizations” (or HROs). He highlights properties such as a preoccupation with failure and a reluctance to simplify interpretations as guardrails for safe autonomous systems. It’s a striking departure from “move fast and break things.”
The lesson of social media and its unintended consequences is not that we should impede progress, but rather progress is more likely when approached with caution and humility. We’re more likely to succeed when we vigilantly anticipate problems.
In a recent interview, the very thoughtful UC Berkeley Professor Michael I. Jordan was asked what is overlooked or not mentioned enough in AI discussions:
The Uncertainty. Really what machine learning has been is ideas from statistics blended with ideas from computer science. But in that blending a few things have been lost. And one of them is worry about the uncertainty. The researchers know about it, but sometimes they don’t focus on it enough. Researchers assume that if you have a huge amount of data, that the algorithm will just output the right answers most of the time.
In ten years, will a slate of AI leaders be dragged before Congress, stripped of their halos, standing bare with only a promise to be more attentive to unintended consequences? “The algorithm will just output the right answers most of the time.” They’ll need a stronger defence.