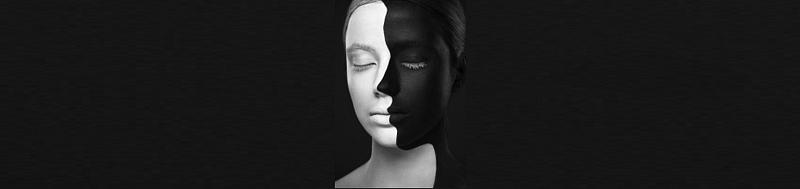
The Pendulum of Progress
Will artificial intelligence revolutionize medicine or amplify its deepest problems?
THE GIST: Ideologies exert a powerful influence in technology and medicine. Although often disguised in modern jargon, belief systems of rationalism and empiricism are ancient. Frequently unexamined, they polarize debates to this day. Yet individually, these ideologies fail to produce workable solutions. The complexities of the real world demand pluralism, the best of both worlds. Today, rebounding from rationalist dominance and fuelled by big data, AI and medicine tend strongly to empiricism. This alignment threatens to entrench the incumbents in cycles of codependency and reinforcing errors. Examples of these cycles include data as a cure-all, theory-free science, and the misuse of statistics. But there’s ample reason for optimism. A range of differences across medicine and AI may bring out the best that each community has to offer: urgency tempered with caution; an openness that values privacy; engineering pragmatism in scientific discovery. Hype and disillusionment are to be expected, a natural part of the process. But this isn’t another AI winter. These pendulum swings and ideological corrections are essential mechanisms of progress.
The American Medical Association (AMA) recently released its first policy recommendations for augmented intelligence. It highlights some of the most serious challenges in artificial intelligence, including the need for transparency, bias avoidance, reproducibility, and privacy. Those working in medicine may find this list familiar. Medicine has long struggled with similar problems.
The similarities are not a coincidence. There are deep philosophical and methodological intersections across AI and clinical medicine. Both professions recently experienced a pendulum swing in their prevailing approaches. And in the zeitgeist of big data, powerful interests in medicine and AI are presently aligned on the same side of a centuries-long ideological struggle.
People are understandably excited about a digital convergence in health tech. But ideological alignment and entrenchment may reinforce these shared challenges in a perverse codependency. The philosophical intersections between AI and medicine are not well known within their respective communities, let alone across them. And this imposes risk. If we’re unaware of the forces that shape our policies and technologies, they may enslave us.
Yet a positive and productive collaboration may unfold. AI and medicine embody important differences that could elevate each side and catalyze innovation. The AMA recommendations are a case in point, presenting technologists with a gauntlet of challenges for positive changes and progress. And as we’ll see, AI has much to offer medicine in return.
How might this new partnership flourish? Will it raise healthcare to new heights or send it on a spiral of mutually reinforcing errors?
Parallel histories of medicine and AI
Throughout their histories, medicine and AI have teetered back and forth between rationalism and empiricism. Peter Markie outlines a struggle between these two systems of thought that extends back to antiquity. “The dispute between rationalism and empiricism concerns the extent to which we are dependent upon sense experience in our effort to gain knowledge. Rationalists claim that there are significant ways in which our concepts and knowledge are gained independently of sense experience. Empiricists claim that sense experience is the ultimate source of all our concepts and knowledge.”
Markie highlights that not even the founders of the respective tribes were strictly one or the other. This message of pluralism remains highly relevant today and we’ll return to it often. Accordingly, we’ll use these terms simply as poles along an axis. Empiricism emphasizes the role of experience, particularly sensory perception, and discounts (or outright denies) the value of a priori reasoning. Rationalism sits at the other extreme. And history swings along this axis as different ideological leanings gain dominance.
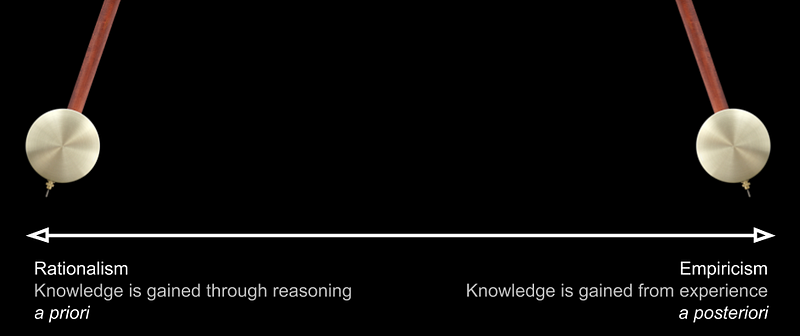
Medicine
Warren Newton summarizes the roots of rationalism and empiricism in medicine. The orthodoxy in the classical age was traditionally rationalist. Rationalists focused on anatomy and the mechanisms of disease, such as the doctrine of humours. Empiricists were splinter groups critical of the authoritative basis of medicine. Empiricists rejected medical theory in favour of observations. Empiricists “based their practice on the recollection of past observations and the knowledge of how similar symptoms had developed, what their outcomes were, and the determination of similarity between the case at hand and previous cases.” If this ancient wisdom sounds familiar, we’ll return to it momentarily in its modern form.
However, the prevailing paradigm at the turn of the 20th-century was rationalist expert-based medicine. The anatomists and proponents of basic science won out. Newton explains, “What we understand as modern medicine has at its roots a triumph of rationalism: the emphasis on the search for basic mechanisms of disease and the development of therapeutic tools derived from them.”
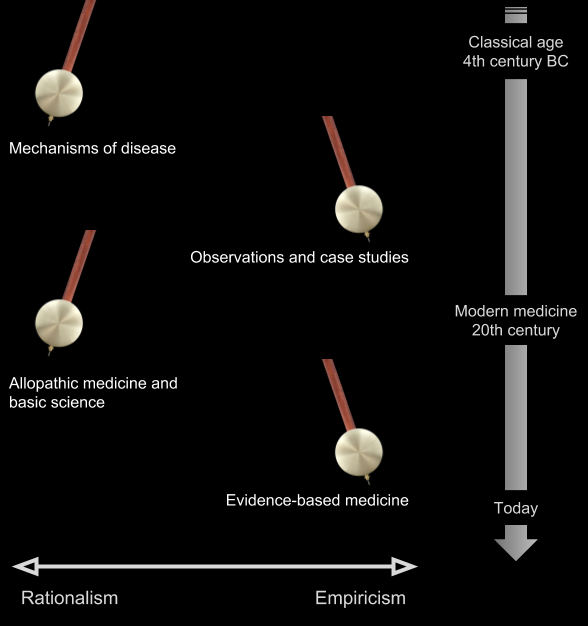
In a seminal article in the 1990s introducing the “new paradigm” of evidence-based medicine, Gordon Guyatt and his collaborators challenged the authoritative basis of expert-based medicine. “The study and understanding of basic mechanisms of disease are necessary but insufficient guides for clinical practice.” Note the conciliatory language and pluralism, necessary yet insufficient.
But the pendulum had swung too far towards rationalism. Science demands criticism through empirical testing, with a toolkit of quantification practices and statistical analyses. A correction was needed. Newton describes evidence-based medicine as “a renaissance of empiricist thinking cast into modern language.” Today, EBM is the prevailing approach to the practice of clinical medicine.
Artificial intelligence
The pendulum of AI swings much faster. In Artificial Intelligence: A Modern Approach, Stuart Russell and Peter Norvig recount a range of empiricist and rationalist approaches. Both symbolic systems of logic and reasoning and connectionist approaches of neural networks and learning demonstrated early promise. But symbolic systems were the first to commercial dominance, as the expert systems in the 1980s. Expert systems combined knowledge-bases and reasoning engines, painstakingly prepared in collaboration with domain experts. In medical applications, this connection between expert systems and expert-based medicine was made explicit.
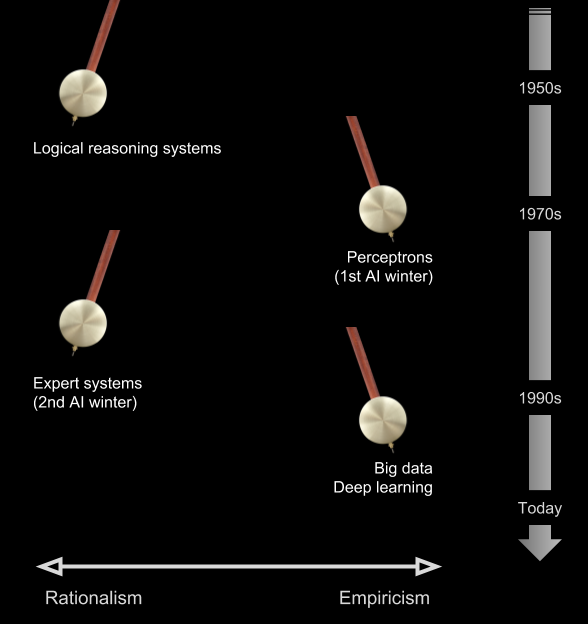
Like expert-based medicine, expert systems proved brittle and their ambitious goals were unrealized. The “authority” of codified expertise in knowledge-bases and rule-based systems was impractical. Quietly, connectionist approaches, with their focus on empirical methods and careful experimentation, gained traction.
Today, machine learning is the dominant form of AI. As I outlined in an earlier post, empiricism is also its dominant philosophy. And as with their predecessors in expert systems, commercial applications of machine learning are reaching deep into medicine.
Cycles of codependency
Pragmatic physicians combine their experience and understanding of biological mechanisms with clinical guidelines validated through experimentation. Similarly in AI. While there’s a healthy tension and natural allegiances, modern AI systems combine elements of empiricist and rationalist approaches. And this is a lesson that’s been repeatedly learned and unlearned: Neither strict empiricism nor strict rationalism deliver workable solutions.
But despite the practical necessity of hybrid approaches, it’s easy to drift into ideological silos. Practitioners of medicine and AI may unknowingly hold strong ideological commitments to empiricism or rationalism, even while applying pluralistic approaches. Blinded by these often unexamined beliefs, empiricists look across the aisle and see Luddites unable to grasp the potential of data-driven AI. Rationalists look back and see an assault on expertise and theory. Ironically, both see in the other anti-scientific sentiments.
And the risk of entrenchment within these ideological silos is real. Prevailing approaches to AI are gaining near monopolistic power in medicine, mirroring the winner-takes-all phenomenon in technology. An independent review panel appointed by DeepMind highlighted risks of monopoly power. While this may be the workings of a competitive market, it may also foreshadow ideological entrenchment, commitments mirrored in long-term investments and policy decisions. We are living in a greenfield moment that may set the course of medicine and AI for decades.
What happens when two industries, moving in unison, amplify their shared ideology? The risk is that both medicine and AI become locked in cycles of codependence and mutually reinforcing errors. Let’s walk through a few examples. The risk is most obvious in the rise of dataism, the first of three cycles we’ll consider.
1. Data as a cure-all
Empiricism has gained momentum in this era of big data. Dataism, a term popularized by the historian Yuval Harari, is empiricism cast into modern language. Dataists view the world as systems of data flows. They “worship data.” In Harari’s terms, information flow is the “supreme value” of this new religion. Today, everything is data-driven (or potentially so). Data-driven has come to mean progress. If you subscribe to dataism, then it follows that access to data and talent are the only factors impeding progress. Central to this creed, any limits on the free flow of data will slow us down. And so legions of dataists rally on social media, defending data as the victim. Data becomes a foundational resource, like oil or electricity, threatened by oppressive policies.
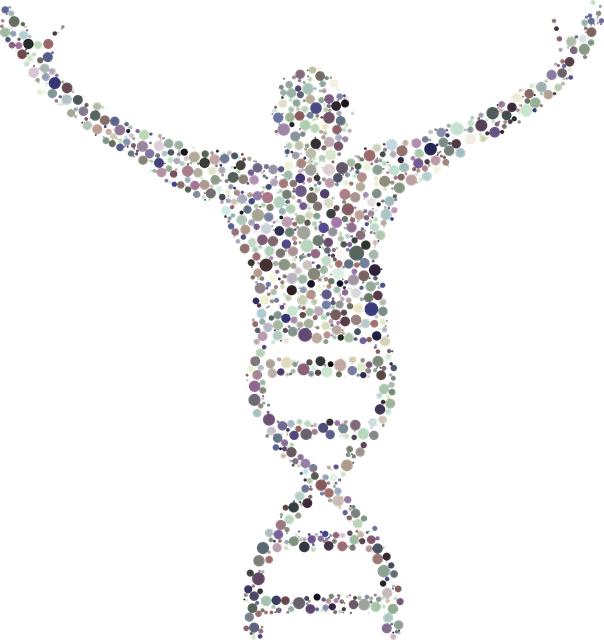
John Nosta warns of the essential role of data, and its real demons. He places data at the core: “Data is the drug. Data is the driver. Data is the dilemma.” And he implores us to embrace progress. “We don’t need a ‘data witch hunt’ where a regressive posture clings to the perception of a safer or better past.” Data proponents acknowledge the risks of privacy and data governance. But these risks are frequently framed as the cost of progress, your data or your life. Nosta concludes, “And there’s no denying that the problem must be managed. But let’s manage the problem without destroying that basis for important and life-saving innovations that tackle those real demons that we call disease.”
Eric Topol and his colleagues at Scripps are also noteworthy proponents of data-driven medicine. They envision a future where a torrent of data from digital medical tools and sensors, the “‘dataization’ of an individual’s medical essence,” leads to a medical revolution in predictive analytics. Situating us in Moore’s law, they ask, “When and how will all this tectonic tech progress finally come to clinical care?” Again, their enthusiasm is tempered by problems of privacy, security and the dehumanizing effects of data-centric doctors.
Of course, proponents like Nosta and Topol are right: Data-driven medicine offers tremendous opportunities and brings significant challenges. But just as access to data is one facet of medicine’s problems, it’s but one facet of AI’s solutions. Elements of dataism, like empiricism, are necessary yet insufficient. Many of the problems with machine learning relate to an over-reliance on data, including issues highlighted in the AMA recommendations. Moreover, new generative approaches, like human beings, are increasingly economical with data. These systems build internal representations of the world based on explanations of their environments, which are then tested using sparsedata. Constraints on data, like constraints generally, drive innovation.
And this pluralism extends into the human aspects of medicine as well, entirely beyond the reach of technology. Benjamin Chin-Yee and Ross Upshur discuss clinical judgement in the era of big data and predictive analytics. They highlight the tensions between different philosophies of clinical medicine, including narrative and virtue-based approaches, and the insufficiency of statistics and machine learning. The moral of our story, “clinical judgement requires a pluralistic epistemology that incorporates and integrates the tools and insights of these different methods.” To reconcile their pluralism with dataism, it’s insufficient to claim that all of life is algorithmic if we don’t know all the algorithms.
And contrary to popular belief, big data can make things worse. In his essay Saving Science, Daniel Sarewitz explains how the empiricism of big data stands opposed to the pursuit of scientific knowledge. “The difficulty with this way of doing science is that for any large body of data pertaining to a complex problem with many variables, the number of possible causal links between variables is inestimably larger than the number a scientist could actually think up and test.” In a moment, we’ll return to this important question of causality and how it complicates data-driven medical research.
These general concerns about data extend to very specific diagnoses and treatments. Adam Cifu and Vinay Prasad highlight how advances in real-time data gathering may lead to worse outcomes if treatments are not well founded. “Even in our era of evidence-based medicine, much of what we do is not based on evidence.” Wearables and smartphones may exacerbate the problem by accelerating the process of diagnosis and treatment. “A new but common trend is the broadening of diagnostic categories based on advances in technology and extrapolating the results of early studies (in more severe disease states) to less severe conditions.” Questionable treatments, more efficiently or pervasively applied, are still questionable treatments.
But dataism doesn’t stop at data. Not only is human expertise discounted, it becomes disposable. Harari connects dataism to the rise of a post-humanism. “The Internet-of-All-Things may create such huge and rapid data flows that even upgraded human algorithms won’t handle it.” Buoyed by the success of machine learning and our data-driven ethic, a particularly stark version of empiricism is taking hold. This is reflected in the next cycle of codependency, theory-free science.
2. Theory-free science
Science is our best exemplar for AI. In many respects, only science captures the truly revolutionary quality of knowledge we expect of AI. Many dream of AI systems that exceed the ability for humans to comprehend their output. This is the promise of artificial generalintelligence (AGI). Proponents of theory-free science are banking on the inevitability of AGI , or at least a range of highly capable narrow AIs, to deliver on this promise. Accordingly, as big data swings the pendulum decisively towards empiricism, theory-free science becomes the wrecking ball of rationalism and human expertise. It’s already bearing down on the expertise of physicians.
Clinical medicine is fertile ground for theory-free science. In their introduction to the philosophy of medicine, Paul Thompson and Ross Upshur maintain that much of medicine “uses theories or models as a unification of current knowledge and the mechanisms underlying the behaviour of things.” Clinical medicine, however, “draws on clinical research, which, currently, is not itself a theory-based or theory-driven domain of medicine.”
That there exists graduations in the quality of scientific evidence is one of the earliest and first principles of evidence-based medicine. Evidentiary hierarchies generally place systematic reviews and meta-analyses at the top, and the expertise expressed in basic science and case studies at the bottom. Deemed overly simplistic, these hierarchical classifications were superseded with more complex assessments of evidence and clinical recommendations, such as the GRADE approach. But the old bastions of rationalism — basic science, physiology, and expertise — remain evidences of last resort.
EBM advocates point to the many cases where expertise failed (that is, where exclusively mechanistic explanations were insufficient and eventually contradicted based on empirical studies). Critics counter with biologically implausible treatments such as homeopathy finding evidentiary support. This uneven record imposes a massive tension on our pendulum. According to Thompson and Upshur, “There’s a growing chorus of clinical researchers, philosophers of science and scientific researchers in other fields who are challenging the lack of emphasis on theory in clinical medicine.” Consider the advocacy of groups such as EBM+. Philosophers such as Jon Williamson and Nancy Cartwright argue explicitly for philosophical and evidentiary pluralism, particularly in the use of mechanistic explanations.
From its inception, EBM has weathered the backlash of its empiricist leanings. In their 25-year retrospective, Guyatt and Benjamin Djulbegovic addressed the most common criticisms of EBM. As expected, it’s frequently decried as anti-science, formulaic, and specifically hostile to expertise. Whatever you think of these criticisms on the merits, it’s important to recognize the ideological reflux. As explained above, these criticisms are expected as rationalists seek to reset the balance.
And again, these concerns are mirrored in AI. Recounting the ancient empiricist and rationalist traditions, Judea Pearl, one of the pioneers of probabilistic reasoning and Bayesian networks, highlights the theoretical impediments to machine learning. Echoing our theme of pluralism, he reminds us that science is a two-body problem. “Data science is only as much of a science as it facilitates the interpretation of data — a two-body problem, connecting data to reality. Data alone are hardly a science, regardless how big they get and how skillfully they are manipulated.” Pearl’s conclusion is that “human-level AI cannot emerge solely from model-blind learning machines; it requires the symbiotic collaboration of data and models.”
I’ve discussed issues of theory-free science and AGI previously. Theory-free science raises questions of inter-theoretic explanations and emergent phenomena. It doesn’t explain how conjectural knowledge, bold leaps of the imagination, will be bridged. It conflates data-as-observations (the stuff of predictions) with theories-as-explanations (the stuff of science). And it’s unclear how we get from here to there, from today’s prediction engines to systems that support dynamic treatments and interventions.
Why does the vision persist? Within both medicine and AI, theory-free science is driven by the problems of complexity and uncertainty. Ahmed Alkhateeb, a molecular cancer biologist at Harvard Medical School, argues the case. Biology, much less the full expanse of nature, is too complex for humans to comprehend. Alkhateeb resurrects the philosophy of Francis Bacon, the father of empiricism. “A modern Baconian method that incorporates reductionist ideas through data-mining, but then analyses this information through inductive computational models, could transform our understanding of the natural world.” Alkhateeb acknowledges the gaps in this vision, principally in the mystery of creativity, observing, “human creativity seems to depend increasingly on the stochasticity of previous experiences — particular life events that allow a researcher to notice something others do not.” But like many visionaries, he sees an incremental path forward in the world of statistics and probability theory.
Dataism and theory-free science may be written off by some as wildly speculative. But the same can’t be said for applied statistics and probability theory, tenets of both medicine and AI. And so, we’ll make one last stop to see how these powerful tools create another cycle of codependency.
3. Applied statistics and probability theory
Both medicine and AI have been rebuked for their inexpert use of statistics and probability theory. Over the past decade, there’s a growing awareness in medicine of these problems. John Ioannidis concludes, “There is increasing concern that most current published research findings are false.” Paul Smaldino and Richard McElreath maintain that the natural selection of bad science defies easy solutions. “Yet misuse of statistical procedures and poor methods has persisted and possibly grown. In fields such as psychology, neuroscience and medicine, practices that increase false discoveries remain not only common, but normative.”
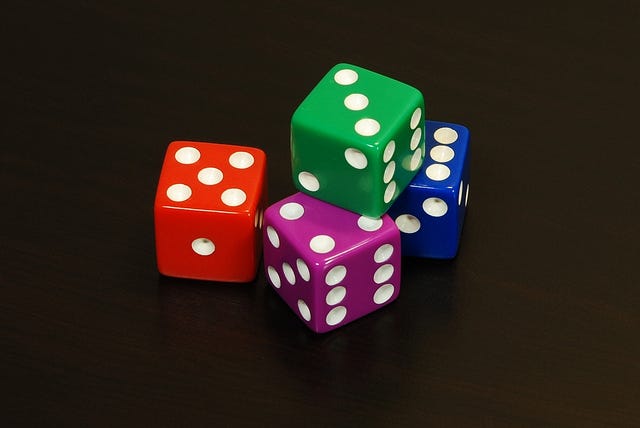
These concerns echo across a chorus of experts. Consider the American Statistical Association’s statement on the misuse of p-values and statistical significance. “The validity of scientific conclusions, including their reproducibility, depends on more than the statistical methods themselves. Appropriately chosen techniques, properly conducted analyses and correct interpretation of statistical results also play a key role in ensuring that conclusions are sound and that uncertainty surrounding them is represented properly.” They conclude with a nod towards rationalism, “No single index should substitute for scientific reasoning.”
In AI, similar concerns are mounting. Pearl has become one of AI’s sharpest critics. Ali Rahimi initiated a storm of debate at NIPS 2017 comparing machine learning to alchemy. Gary Marcus added a scathing critical appraisal of deep learning. There have been high profile failures, such as Microsoft’s racist Tay experiment and IBM’s disappointments in cancer care.
The misuse or over-reliance on statistics and probability theory is a recurring theme. Previously, I discussed the conflation of prediction and explanation and how the indiscriminate use of statistical modelling has reached epidemic proportions. It’s difficult to overstate the importance of these distinctions in medicine and the need for a judicial use of these tools. People need more than descriptions of their ailments or assessments of risk. They need interventions and treatments. Yet many prospective applications of AI do not have the flexibility to incorporate interventions and treatments.
And there’s a well-intentioned (and highly lucrative) effort to further democratize AI for the masses, through shared data, open source software and cloud infrastructure. The combination of economic incentives and ease-of-use brought a productivity explosion in both AI applications and medical research. But it doesn’t always lead to solutions that are congruent with the problems.
In these highly complex and uncertain environments, visionaries from both medicine and AI are banking on the ability to extract reliable causal knowledge from statistics. Vijay Pande, the former director of the biophysics program at Stanford University and a general partner at VC firm Andreessen Horowitz, is one such visionary investor. Invoking one of technology’s central ethics, he describes the democratization of healthcare. “Like the best doctors, AI can constantly be retrained with new data sets to improve its accuracy, just the way you learn something new from each patient, each case. But the unique ability of AI to apply time-series methods to understand a patient’s deviation from baseline on a granular level may allow us to achieve a statistical understanding of causality for the first time.”
Unfortunately, it’s not at all clear how this statistical understanding of causality will be realized. Thompson and Upshur maintain that “determining causality without a theory or model is elusive.” On the role of theory and evidence in medicine, Mita Giacomini raises similar concerns about theory-free methods and their reliance on statistics and probabilities. “The RCT has inadvertently become the new ‘Emperor with no clothes.’ Attired in sophisticated methods, RCT evidence is sometimes welcomed as authoritative even when it is incapable of providing meaningful information — particularly, when the underlying causal theory is inscrutable.”
These questions are repeated across AI, philosophy and science. In The Book of Why, Pearl recounts a personal journey of discovery. “The recognition that causation is not reducible to probabilities has been very hard-won, both for me personally and for philosophers and scientists in general.” Yet the cycles of codependency spiral on. “Every time the adherents of probabilistic causation try to patch up the ship with a new hull, the boat runs into the same rock and springs another leak.”
The boat runs into the same rock, whether it sails from medicine or AI. Tools may be misapplied and roadmaps may anticipate progress that’s slow to emerge. Yet if both sides maintain that the gaps in our knowledge will be solved incrementally, or worse, if those gaps are entirely denied, errors may be amplified.
From hype to progress
The common struggles across medicine and AI follow from their philosophical and methodological intersections. As such, we could go on and on, illuminating cycles of codependency and opportunities for mutually reinforcing errors. But I’d rather conclude with a more positive and hopeful perspective. There’s a tremendous opportunity for AI and medicine to be mutually elevating.
“Moments such as this often signal the beginning of a conceptual revolution.” — Paul Thompson and Ross Upshur
Cycles of hype and disillusionment are nothing new in technology. And as Chin-Yee and Upshur explain, it plagues medicine, as well. “Enthusiastic endorsements of big data and predictive analytics are not limited to the popular media but are also voiced by leaders of the medical community and abound in the medical literature, including many of the field’s most prominent journals.” Once hype is credentialed in this way by leaders in both domains, it becomes entrenched. As Nosta puts it, “The big data toothpaste is out of the tube and there’s really no putting it back, however bad that taste might be.”
But the pendulum won’t resist its corrections indefinitely. Gradually it changes direction, first pacifying the incumbents, then moving with conviction as new tribes gather and organize. There’s a tendency to view these corrections as “going back” to a previous time and it’s a valid concern. We should vigilantly defend progress. But corrections also combat ideological entrenchment and dogma. Despite the consternation and worry of AI proponents, criticism doesn’t foreshadow failure, it makes room for progress.
And progress may emerge quickly. One thing that AI brings to the table, unparalleled in medicine, is speed. Where the rate of progress in medicine is measured in years if not decades, in AI it’s measured in weeks. Technologists are able to experiment at a rate that would be unconscionable in the life-and-death world of medicine. In virtual worlds, AI provides safe environments to confront their common technical challenges. Innovations can thereafter be safely directed back into medicine.
Yet at the same time, it’s important to remember that speed kills. Unlike the more abstract domains of advertising and marketing, medicine deals in reality, and reality kicks back in the worst ways. The Silicon Valley ethic of “move fast and break things” is not welcome in medicine. I’m reminded of the wisdom of the biologist E. O. Wilson and his book Consilience, The Unity of Knowledge, incidentally the book that drew me into artificial intelligence. “In ecology, as in medicine, a false positive diagnosis is an inconvenience, but a false negative diagnosis can be catastrophic. That is why ecologists and doctors don’t like to gamble at all, and if they must, it is always on the side of caution. It is a mistake to dismiss a worried ecologist or a worried doctor as an alarmist.” Or as a Luddite.
Medicine provides an environment where the known problems and limitations of machine learning cannot be tolerated, and the AMA should be applauded for their advocacy. It provides a catalysis for innovation in AI. Much has been said of the their decision to reframe AI as augmented intelligence, not artificial intelligence, to emphasize a subordinate role for these technologies. This is a clear-headed assessment of the present limits of AI. It’s a position of pragmatism and pluralism, not Luddism.
Collaborations in AI may also promote the shift to open science. Although there are differences in aspects such as patient privacy, the AI community will not gently forgo the values of openness, even if opposed by the medical establishment. Witness the AI boycott of the closed-access journal, Nature Machine Intelligence. Similarly, medical research and much of science generally have been criticized for lacking direction and accountability. Sarewitz maintains that “scientific knowledge advances most rapidly…when it is steered to solve problems — especially those related to technological innovation.” He concludes, “Only through direct engagement with the real world can science free itself to rediscover the path toward truth.” AI exemplifies this type of technology-driven, pragmatic problem solving.
A recurring theme in this discussion is the need for pluralism, to draw on both empiricist and rationalist ideas. Thompson and Upshur remind us of the risks of entrenchment and vested interests. “Indeed, the vast majority of clinical researchers, regulatory agencies and clinical practitioners either reject, ignore or are oblivious to these criticisms. We attribute this to entrenchment but there are obviously other elements. Moments such as this often signal the beginning of a conceptual revolution.” Summoning Charles Darwin, they look to young and rising clinical researchers “who will be able to view both sides of the question with impartiality.”
What we’re witnessing in medicine and AI is the pendulum swing of progress. Data and observations are balanced with theory; machine-based solutions are balanced with human expertise; statistics and probabilities are balanced with causal knowledge. These tensions and debates, difficult as they seem from within, signal progress not failure. And they’re essential to it.
Alkateeb, A. (2017). Science has outgrown the human mind and its limited capacities. https://aeon.co/ideas/science-has-outgrown-the-human-mind-and-its-limited-capacities
Chin-Yee, B. & Upshur, R. (2017). Clinical judgement in the era of big data and predictive analytics. Journal of Evaluation in Clinical Practice. https://doi.org/10.1111/jep.12852
Cifu, A. & Prasad, V. (2016). Wearables, smartphones and novel anticoagulants: we will treat more atrial fibrillation, but will patients be better off? Journal of General Internal Medicine 31: 1367. https://doi.org/10.1007/s11606-016-3761-8
Clarke B., Gillies D., Illari P., Russo F. and Williamson J. (2014). Mechanisms and the evidence hierarchy. Topoi 33(2): 339–360. https://doi.org/10.1007/s11245-013-9220-9
Giacomini, M. (2009). Theory Based Medicine and the Role of Evidence: Why the Emperor Needs New Clothes, Again. Perspectives in Biology and Medicine. Johns Hopkins University Press. https://europepmc.org/abstract/med/19395822
Harari, Y. N. (2016). ‘Homo sapiens is an obsolete algorithm’: Yuval Noah Harari on how data could eat the world. https://www.wired.co.uk/article/yuval-noah-harari-dataism
Markie, P. (2017). Rationalism vs. empiricism. https://plato.stanford.edu/archives/fall2017/entries/rationalism-empiricism/
Newton, W. (2001). Rationalism and empiricism in modern medicine, 64 Law and Contemporary Problems 299–316 (Fall 2001) https://scholarship.law.duke.edu/lcp/vol64/iss4/12
Nosta, J. (2018). Data and its real demons. https://www.forbes.com/sites/johnnosta/2018/04/03/data-and-its-real-demons
Pande, V. (2018). How to democratize healthcare: AI gives everyone the very best doctor. https://www.forbes.com/sites/valleyvoices/2018/05/23/how-to-democratize-healthcare
Pearl, J. (2018). Theoretical Impediments to Machine Learning With Seven Sparks from the Causal Revolution. https://arxiv.org/abs/1801.04016
Pearl, J. & Mackenzie, D. (2018). The book of why: the new science of cause and effect. Basic Books
Russell, S., & Norvig, P. (2009). Artificial intelligence: a modern approach (3rd ed.). Pearson.
Sarewitz, D. (2018). Saving science. https://www.thenewatlantis.com/publications/saving-science
Thompson, R. E & Upshur, R. E. G. (2017). Philosophy of medicine, an introduction. Routledge.
Topol, E. J., Steinhubl, S. R., Torkamani, A. (2015). Digital Medical Tools and Sensors. JAMA. 2015;313(4):353–354. https://doi.org/10.1001/jama.2014.17125