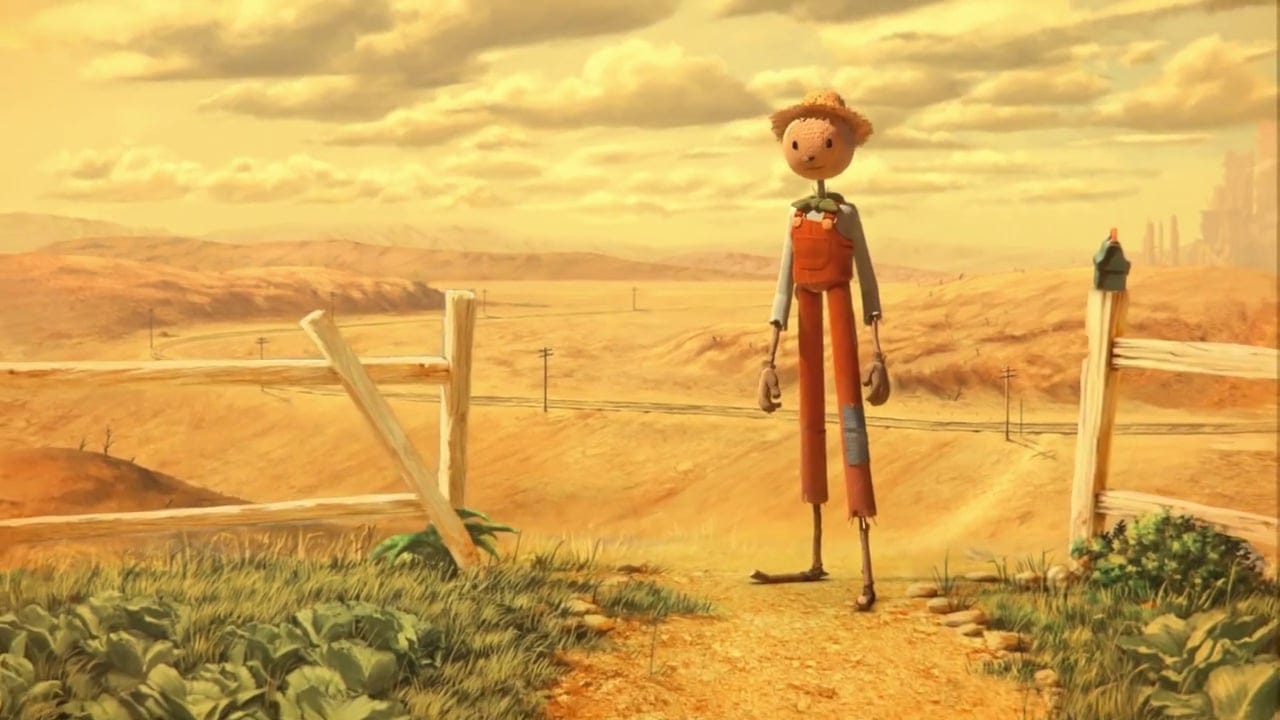
The Yellow Brick Roadmap
Is human intelligence a roadmap for AI?
Every great project needs a roadmap. For many, the path to AI entails a deep understanding of human intelligence. If that seems obvious, you’ll be surprised how this story unfolds and the key role your expertise may play in it.
I’ve argued that human intelligence is bloatware, and bloatware can kill (or at least seriously delay) even the most determined projects. The exemplar of superhuman intelligence isn’t human intelligence, it’s the human invention of scientific discovery.
But even if you agree that automated scientific discovery is the right goal, isn’t a deep understanding of human intelligence prerequisite knowledge? Aren’t the most foundational ideas of neural networks and deep learning derived from this roadmap? And if not, what are the alternatives?
In this post, I’ll examine the arguments behind the human intelligence roadmap and why it should be treated with skepticism. I’ll also surface the key question for evaluating the merits of your own roadmap.
Is human intelligence our best and only example?
Jeff Hawkins, one of the most committed proponents of biology directed AI, opens his excellent IEEE Spectrum essay with the question, Why do we need to know how the brain works to build intelligent machines?
“The only example of intelligence, of the ability to learn from the world, to plan and to execute, is the brain. Therefore, we must understand the principles underlying human intelligence and use them to guide us in the development of truly intelligent machines.” — Jeff Hawkins
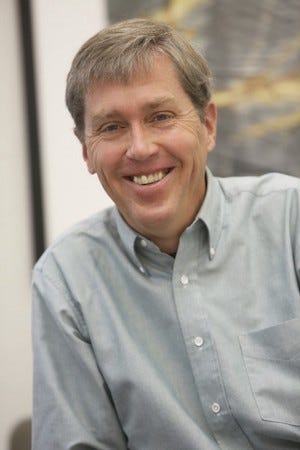
It’s a succinct answer, but the question deserves deeper consideration. The human brain is most certainly not our only example of intelligent systems. Humans (and other animals) exhibit much greater intelligence in networks. These networks exhibit emergent properties quite unlike the individual entities, and critically, they are understood through vastly different explanatory frameworks. There are many other models of intelligent systems and crowd wisdom to consider, such as the swarm intelligence of insects, prediction markets, our institutions, and social media.
Nor is human intelligence our best example of knowledge creation. Our most celebrated process, the scientific method, isn’t a natural phenomenon at all, but rather a human invention.
Isn’t this obvious?
Obviousness is perhaps the most pernicious argument. It’s remarkable how often the terms, simple or obvious, travel with this question. Given that artificial general intelligence doesn’t exist, it’s exceedingly difficult to imagine what form it might take. The analog of human intelligence rushes into this vacuum; pause before you rush in after it.
The abstraction of knowledge creation from the various embodiments of that process, whether natural or mechanical, is deeply non-obvious.
The abstraction of knowledge creation from the various embodiments of that process, whether natural or mechanical, is deeply non-obvious. Consider a much simpler historical example: Imagine how difficult it was to envision flight without thinking of birds or boats, before you observed it in airplanes and rockets. We look back at these imaginings of mechanical flight with a smirk, but history will be no kinder to claims that the human-machine analog is obvious.
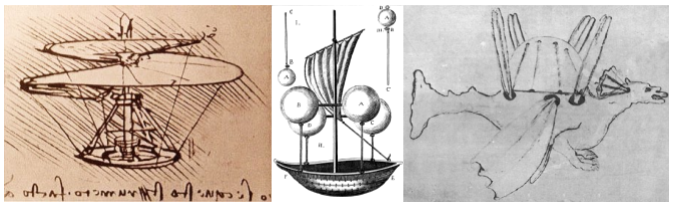
Isn’t this prerequisite knowledge?
You may be inclined to the argument, We can’t proceed without a detailed understanding of how humans think. It’s our prerequisite knowledge.
There’s clearly much to learn about the machinery of natural intelligence. This is the pursuit of good knowledge, knowledge that could inform how to build intelligent machines. Yet only some of our knowledge of natural intelligence may apply to the goal of building knowledge creating machines. Hawkins explains:
“From the earliest days of AI, critics dismissed the idea of trying to emulate human brains, often with the refrain that ‘airplanes don’t flap their wings.’ In reality, Wilbur and Orville Wright studied birds in detail….In short, the Wright brothers studied birds and then chose which elements of bird flight were essential for human flight and which could be ignored. That’s what we’ll do to build thinking machines.” — Jeff Hawkins
But is human intelligence the right prerequisite knowledge? As highlighted above, knowledge creation is our goal, and knowledge creation takes place in different and emergent strata, far beyond the mechanics of individual brains.
Hoping to build a knowledge creating machine without a theory of knowledge is akin to the faith that a machine with wings will fly, without any explanation of how.
Rather, it is a theory of knowledge that is analogous to the theory of flight. Hoping to build a knowledge creating machine without a theory of knowledge is akin to the faith that a machine with wings will fly, without any explanation of how. As an archetype for knowledge creation, human intelligence is a bird that fails to fly at a spectacular rate.
The proof is in the pudding
Proponents of biology directed approaches point to the successes of their approach as evidence. In his essay, Hawkins identifies several concrete discoveries from natural intelligence — learning by rewiring, sparse distributed representations, and sensorimotor integration.
But is anyone debating the contributions of cognitive science to informmachine intelligence? The question is whether human intelligence provides an efficient roadmap. I can frame the functionality of a prediction engine as both a component of human intelligence and a component of the scientific method. Which is the more efficient frame on the path to automated scientific discovery?
Hawkins himself asserts that the connection between machine intelligence and neuroscience is tenuous at best:
“Isn’t most of AI built on ‘neural networks’ similar to those in the brain? Not really. While it is true that today’s AI techniques reference neuroscience, they use an overly simplified neuron model, one that omits essential features of real neurons, and they are connected in ways that do not reflect the reality of our brain’s complex architecture.” — Jeff Hawkins
In truth, most of the innovations in machine intelligence are designed, not evolved solutions. This is hardly an effective appeal for biology directed approaches! And the breakthroughs that Hawkins celebrates, such as sparse representations, seem novel only if you’re rooted to the conceptual frame of human intelligence. In the more generalized context of information, not so much.
Deliberating over the twists and turns in the arguments above, is it likely that human intelligence is an efficient roadmap? More importantly, what are the opportunity costs in the roads not taken?
The fundamental question to direct your roadmap
Nature is a misleading guide for intelligent machines and so are our natural intuitions. There is nothing obvious or predestined about human intelligence as a roadmap. Just as human intelligence is bloatware, the study of human intelligence is no substitute for a roadmap that’s laser focused on the right goal.
The study of human intelligence is no substitute for a roadmap that’s laser focused on the right goal.
Much of the machine intelligence complex seems to drift from this fundamental proposition: The goal isn’t human intelligence, it’s knowledge creation. It may be the case that we’re unable to traverse the distance to artificial general intelligence without a deep and thorough understanding of human intelligence. But the destination isn’t learning or prediction or consciousness or any other component of natural intelligence, whole or in part. Our goal is a machine capable of creating revolutionary scientific knowledge.
And this pursuit may be informed by areas quite distant from the study of human intelligence. For many, the belief that cognitive science is a prerequisite to any meaningful contribution in machine intelligence is paralysing. This is a devastating problem. The magnitude and importance of machine intelligence calls everyone. The effort may draw as much from philosophy and the social sciences as it does from computer and cognitive sciences.
We can’t just shut up and predict; data and observations are insufficient. Reverse engineering human intelligence may capture the popular imagination, but as a roadmap, it’s inefficient at best and a boondoggle at worst.
In crafting your roadmap, start with this fundamental question: What is the underlying theory of knowledge creation that will give your project flight? What is the explanation for why your machine will create good knowledge?