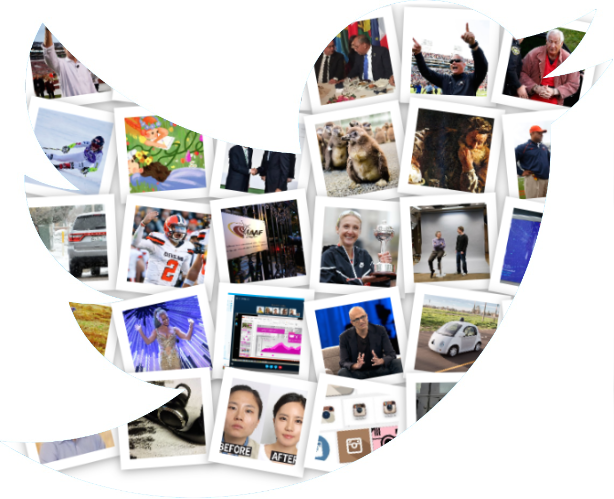
The Surprising Potential of a De-Smooshed Twitter
A unique real-world experiment, powered by an AI, that highlights the rewards and challenges of treating people on Twitter like individuals.
Twitter is a social network and an interest network. It blends experiences that are based on individual interests (such as hashtags and Moments) with social experiences (such as conversations and timelines).
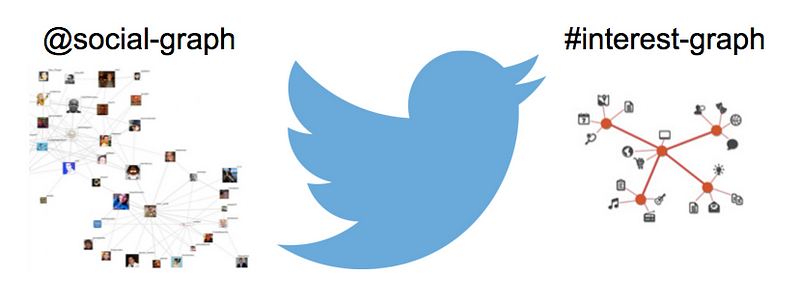
Much of the criticism and hand-wringing over Twitter can be distilled to what Robinson Meyer refers to as smoosh: the tensions that arise when different forms of communications and information collide within the same network. According to Meyer:
The public knows about conversation smoosh, and that constitutes, I think, a major problem for Twitter the Company. New products like Moments — which collects tweets, images, and video into little summaries — are not going to fix that.
I’m not sure anything can fix it, honestly. But I wonder if Twitter can’t arrange a de-smooshing, at least a little bit, by creating more forms of private-ness on the site.
Create “more forms of private-ness on the site.” Meyer cautions about the lack of data in this area to support his intuitions, a gap we hope to address with this report. It’s based on a unique real-world experiment, powered by an AI, that de-smooshes, or separates, the social aspects of Twitter from those that cater to “private-ness” and individuality.
In the sections below, we summarize how we’re approaching our experiment, what we’ve learned about individuality on Twitter, the appetite for individualized services, and the degree to which Twitter, as a social medium, supports services that cater to individuals.
Lastly, we offer some concrete recommendations for how social and individual experiences of Twitter can peacefully coexist. It’s a glimpse into one possible future of Twitter where the boundaries between social and interest networks are clarified, and the potential of each more fully realized.
Some of our more surprising findings that recommend individuality and interests as a growth engine within Twitter:
- People on Twitter act like individuals. They express widely divergent interests.
- People on Twitter want to be treated like individuals. There is strong demand for individualized services, evident in uncommonly high rates of organic growth.
- Services that cater to individuals are more engaging, on a per-follower basis, than services that cater to larger groups of people.
- The social graph is a fragile basis for recommending content. Content recommendations based on social connections are much more likely to prompt an unfollow than recommendations based on individual interests.
- It isn’t sufficient for content recommendations to be objectively relevant to an individual’s interests. They must also be consistent with that individual’s self-expression, how they want to be presented in a social context.
- Social services seek to maximize impressions; individualized services seek to narrowly target impressions. This tension is the single greatest challenge to running individualized services within a social medium like Twitter.
- While increasing rates of activity on Twitter can fuel growth, the diversity of interests across social networks impose an offsetting, dampening effect.
- There’s a single organizing basis that’s necessary yet sufficient for matching information to individual interests: semantic data that describes the interests expressed in tweets and the recommended content items.
Report Summary
Recommendations for Marketers and Service-Providers
Despite the tendency of data and analytics to obscure our differences, Twitter is very much a network of individuals.
There are tremendous, untapped opportunities within Twitter for those services that can effectively cater to individuals and niche audiences.
The value proposition explored in the @Primal experiment, personalized content recommendations, may act as an umbrella for an unbounded number of new services, each targeting specific informational needs and jobs. There’s a healthy appetite for these services within Twitter and a remarkable amount of growth, engagement, and goodwill to be earned for the effort.
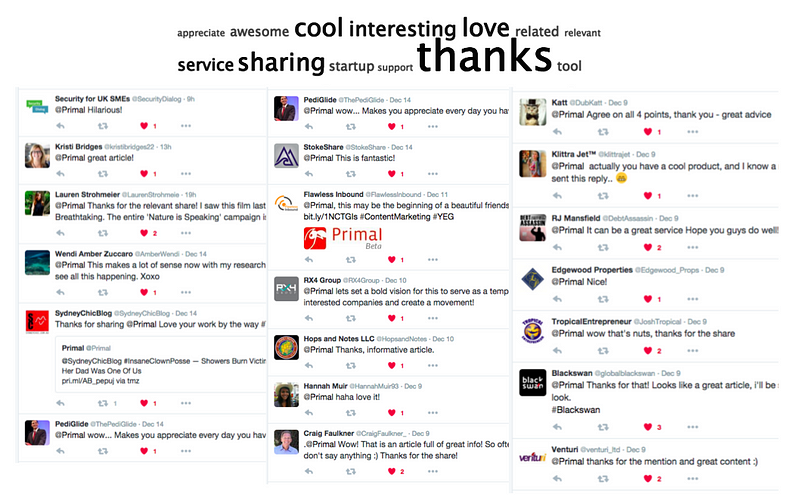
There are also, however, systemic challenges in pursuing these opportunities within Twitter. Twitter is a social network to the core. Regardless of the many jobs it may support, it is first and foremost a medium for socializing across large groups of people.
In catering to individuals, marketers and service-providers need to be aware of this social context, that their service will become part of the larger social expression.
As a reflection of the individual, the stakes are much higher than for services that cater to large groups.
Success hinges on the ability to precisely target your information to the right audience. Twitter’s social graph is supportive of it, but you need to be aware of the various ways the social graph blurs the boundaries of individual interests in ways that may undermine your targeting efforts.
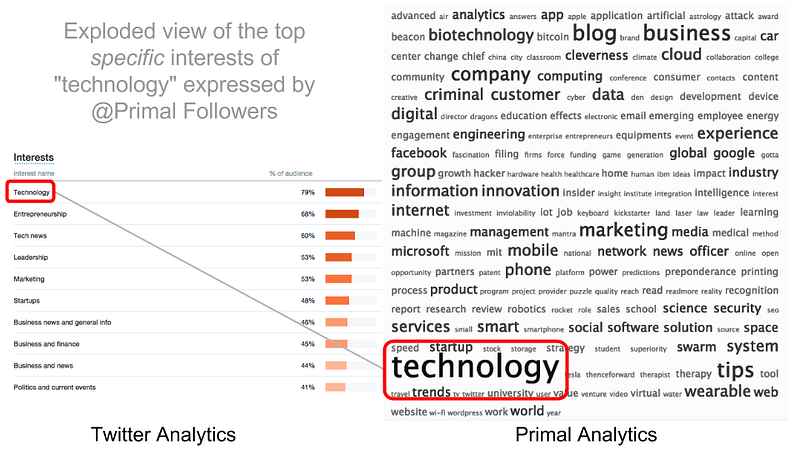
However, if supporting data can be brought to bear, produced by a community of curators (at small scales) or machine intelligence (at large scales), Twitter provides tremendous opportunities for services that cater to individuals.
Recommendations for Twitter
In the spirit of Twitter’s #helloworld solicitation for suggestions, we have the following recommendations, one bold and one modest.
To be bold, few things as complex as Twitter have a silver-bullet solution. Twitter, however, does: it should decouple the interest graph from the social graph.
Some would argue that Twitter is in a state of decay, when it’s really only in a state of disorganization.
A social network and interest network can coexist, but only insofar as the users and services that operate on this compound network remain oriented as they express their social and interest-based intentions.
Many solutions proposed to Twitter involve compounding an already compounded network with more organizing bases, such as adding another layer of curation or another source of data. As recommended above, these strategies are necessary to segment niche audiences when working outside of Twitter, but Twitter can tackle the root of the problem from within.
The root of the problem is that Twitter smooshes the social graph and interest graph in unpredictable ways.
Fortunately, the hooks for expressing interests and social relationships already exist within Twitter, via hashtags and mentions. What’s presently lacking is a precise, user-directed way of targeting information within these bases, particularly with respect to interests.
How? Treat the # as the @. Just as tweets can be targeted within the social graph by beginning the tweet with a username, targeting within the interest graph could follow the same convention, beginning the tweet with a hashtag.
Critically, this decoupling must extend down to the algorithmic level, where more precise interests-based targeting is supported by fine-grained interest graphs. Provisioning more data about interests would allow Twitter to identify small fluid communities of interests that don’t need persistent social connections to work effectively. Like Moments-for-individuals, users can express and follow their interests without the additional burden of identifying users that share their interests.
If this recommendation seems audacious, consider the benefits:
- New users would not need to build a social network to immediately gain value from Twitter’s interest network, eliminating the cold start problem.
- Existing users would not need to curate their social network to keep it aligned with their evolving individual interests (unless they wanted to).
- Casual users and ad hoc communities of interest could flourish, without the overhead of needing to form and disband social connections.
- Marketers and advertisers could accomplish their goal of more precise targeting of content and messaging, without needing to divine imprecise social expressions of interests.
- If the size of its latent interest graph is any indication, Twitter would seize a growth opportunity that is considerably larger than any social network.
Decoupling the social graph from the interest graph is our bold recommendation. Here’s a modest request: Twitter’s usage data and analytics are generous and a huge draw among product innovators. But real innovation demands more fine-grained metrics that capture new product values and success criteria. And more importantly, metrics that report on our failures and missteps are more valuable than any positive reinforcements you could provide.
Background: Why Are Individuals So Important to Twitter?
As a social network, Twitter caters to large groups of people, but it also serves individuals and their unique interests. A huge push in this direction was in their shift from social network to interest network, circa 2012:
Twitter connects you to things you care about. We think of Twitter as more of an interest network instead of a social network.
Twitter connects you to the things you care about. It’s a key area of competitive differentiation. Compare the purpose of Facebook, a more unambiguously social network, which “celebrates how our friendsinspire us, support us, and help us discover the world when we connect.”
But Twitter never abandoned its social roots. Unlike its competitors, Twitter elected to compound (or smoosh) the social and interest-based experiences, as opposed to separating them across distinct media (such as Facebook, the social network, and Facebook Messenger, the messaging platform).
This focus on individuals and interests has become a mainstay of the Twitter experience, evident in how they onboard new users and in features like tailored suggestions, highlights and Moments. Why?
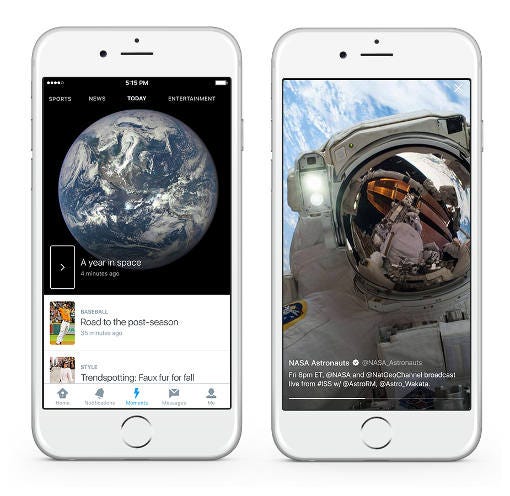
Advertisers and marketers are clearly demanding targeting based on interests. They want to precisely target their content and messaging to the right audiences. They want an aggregation of individuals, specifically those individuals that will find their content and messaging compelling.
But perhaps most importantly, there is a large contingent of people in social media that need more individually tailored services because social media is failing them. New users, casual users, inactive users, neglected users are all terms that describe segments of individuals that are outside of Twitter’s core.
Ultimately, the long tail of individuals represents massive growth opportunities for Twitter.
Part 1: About The Experiment
It’s notoriously difficult to run experiments in complex social environments. There are just too many variables to control. Intelligent machines have an important role to play in this regard. Their behaviour can be tightly constrained, simplifying one side of the experiment. They allow us to systematically vary the environment to tease out more information about the social interactions.
Our vehicle for this experiment is @Primal, a personal intelligent assistant for content discovery, or ant for short.
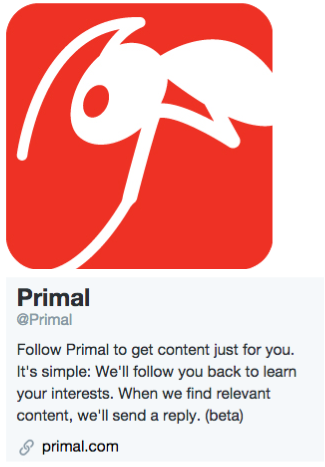
To use the service, simply follow @Primal. Our ant will follow you back to learn your interests from your tweets. If it finds content relevant to your interests, it tweets back a reply.
For the past 8-months, we’ve been running a public beta of the service. Our beta began in May 2015 and is ongoing. Over this time, the number of followers participating has grown from less than 500 to over 16,800. Over that same period, Primal has recommended content in over 140,000 tweets.
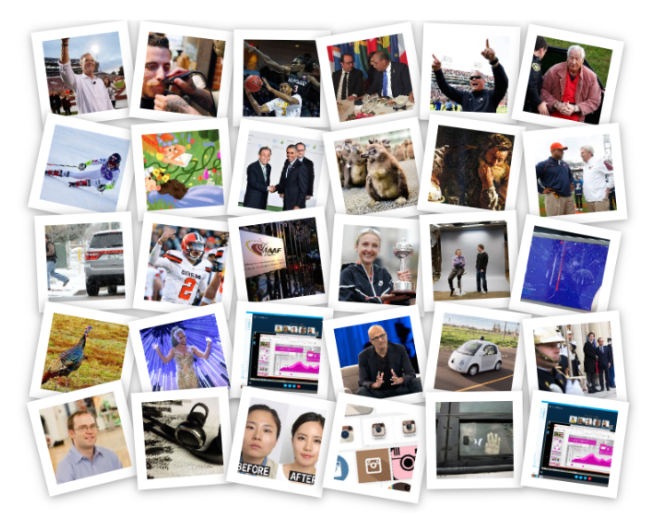
A Service that Caters to Individuals
While content discovery services are common on Twitter, what sets this service apart is its focus on individuals. We don’t broadcast our tweets to all our followers at once. Rather, we send a unique reply to each individual follower, catering to the interests they express in their tweets and retweets.
A reply is a special type of tweet that is targeted to a specific recipient. According to Twitter, “When a tweet starts with a @username, the only users who will see it in their timeline (other than the sender and the recipient) are those who follow both the sender and the recipient.”
In order to avoid contacting followers too frequently, we limit the service to not more than one daily reply per follower. Due to constraints on the number of tweets we can send in the aggregate, discussed in detail below, the actual productivity of the service is lower.
Every content item selected, every tweet sent, is uniquely constructed for a single recipient. We’re so committed to this aspect of individuality that we avoid sending the same content item to anyone more than once.
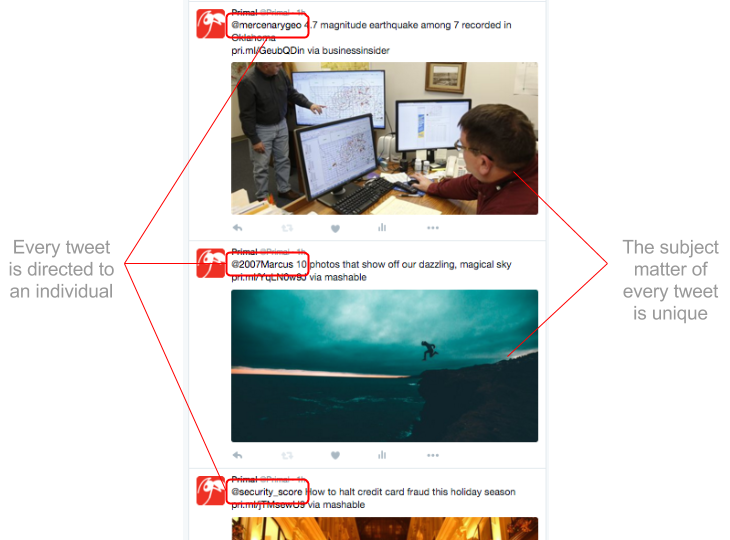
How Primal Works
People treat each other like individuals all the time, but it’s challenging to scale an individualized service up to even hundreds, let alone thousands or millions of people. Automation and machine intelligence are key.
The graphic below illustrates how Primal operates. We’re using Twitter’s Streaming API to learn the interests of our followers. Our artificial intelligence creates an interest graph representing either an individual tweet or a collection of their recent tweets (depending on their relative suitability). It then organizes content from the web based on their interests. If the content is relevant enough, the ant replies to the user with the title of an exemplary article and a link to read more.
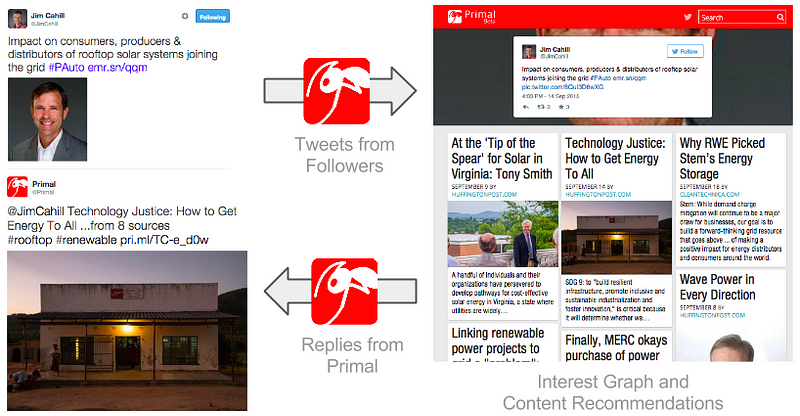
Using An Intelligent Assistant to Run A Controlled Social Experiment
Bots have a well deserved reputation as the scourge of social media. So just to get out in front of any objections to the idea of using an intelligent assistant as the vehicle for this experiment, these aspects make Primal’s ant different from bots:
- Most bots exhibit no intelligence. Within the narrow confines of its job (its speciality), Primal’s ant is intelligent. As outlined in more detail below, in many ways Primal’s ant outperforms people in the task of personalized content discovery, and people often assume that Primal is powered by people, not a machine.
- Bots are often very simple programs. Primal’s ant is powered by a proprietary artificial intelligence built through over 10-years of effort, more than $20-million in funding, and disclosed in over 120 patent filings.
- Bots rarely tailor their behaviour to individuals. Even those that are capable of reacting to unique inputs, such as chat bots, typically draw from the same general domain or process knowledge. Primal’s ant is following the same basic process for all its interactions (that is, content discovery and delivery within Twitter), but it’s underlying knowledge is generated specific to each and every individual user.
What Primal’s ant has in common with bots is that it is a fully automated machine. While this aspect is essential for building individualized services at scale, machines occasionally make recommendations that don’t make sense to people. Fortunately, these missteps also surface important information for this experiment, as we’ll explore below.
Part 2: Do Twitter Users Want Services for Individuals?
While it might seem obvious on the surface of it that everyone wants to be treated as an individual, it’s less obvious whether everyone wants to be treated that way on Twitter, a social medium.
We evaluated this question through a number of different frames: measures of demand, growth rates, engagement rates, and user sentiments. Perhaps most importantly, we evaluated whether people on Twitter act like individuals at all.
Is there High Demand for Individualized Services?
The first question we investigated is whether there is significant demand among Twitter users for a service targeted to individuals.
Note that this question may be evaluated separately from the performance of our service. We address our performance later. Here, we’re focusing on whether people desire individualized services in the first place, a question that’s relevant to all marketers and service-providers.
How We Tested the Demand for Individualized Services
Our expectation was that, if there is significant demand for a service for individuals, we should see exponential growth in the number of new followers (net of “unfollowers”).
We measure growth in compounded weekly growth rates (that is, growth that is measured in terms of the previously measured week). The strength of the demand will be reflected in the size of the compounded growth rate.
Further, if the appeal is universal across Twitter, we would expect that growth to be extremely uniform, at least so long as Primal’s ant is behaving in a uniform, consistent way. In contrast, a service that appeals to a niche audience would be expected to experience more erratic growth (as the sample in any given period hits or misses this niche audience).
To evaluate the demand, we crafted a simple statement of the value proposition for the bio in our Twitter profile:
“Follow Primal to get content just for you.”
Not everyone takes the time to review a profile before making a decision to follow. Therefore, we further expect that people will follow Primal if they have an interest in the content items we’re sharing with our followers (without necessarily reviewing our bio). That is, we consider “content that matches my individual interests” to be the implicit description of an individualized service.
Given that we started this beta with less than 500 followers, we did not expect there to be any significant contribution of brand recognition to our follower growth. We further controlled our evaluation of the demand by avoiding any additional promotions of the service: we did not advertise the service or otherwise invest in promotions to inflate the demand.
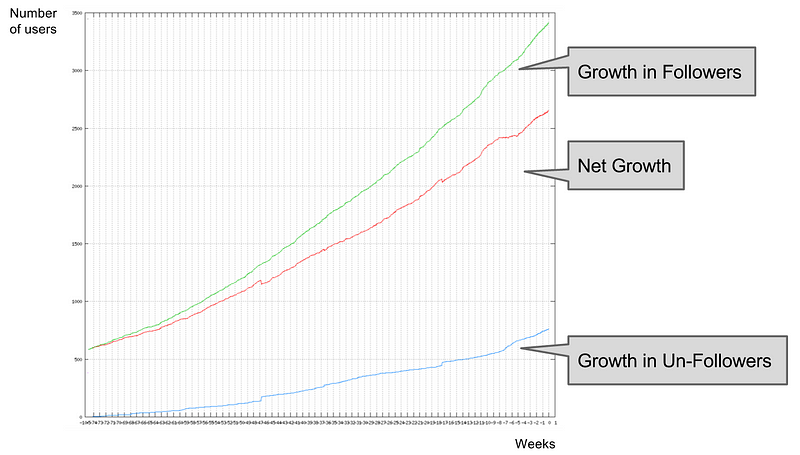
What We Learned About the Demand for Individualized Services
Following the guidance of Paul Graham, we assumed a compound weekly growth rate of 5–7% would be considered good, 10% would be considered excellent. Against this measure, we concluded that there is strong demand on Twitter for content discovery for individuals.
We evaluated the demand over a 2-month period of uniform behaviour in the ant. Over this time horizon, the compound weekly growth rate averaged 13%.
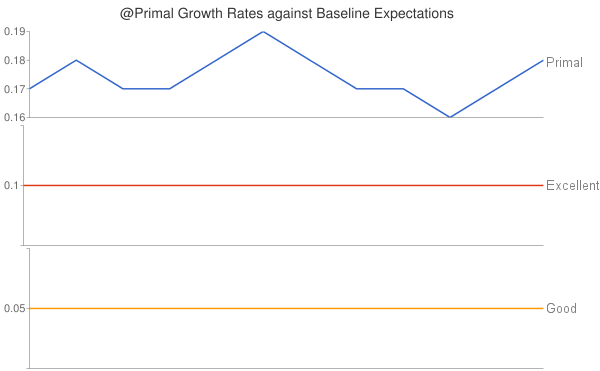
Perhaps more important than the absolute size of the growth rates is their consistency: The rates varied between a narrow range of 12–15%, indicating that this demand may be quite pervasive across Twitter (as opposed to representing a small niche opportunity).
That said, Primal’s followers are certainly a niche of early-adopters, with interests in technology, entrepreneurship and marketing disproportionately represented. Curiosity in Primal as a vehicle for automated and personalized marketing may be contributing to the high rates of growth, beyond what’s promised in its value proposition.
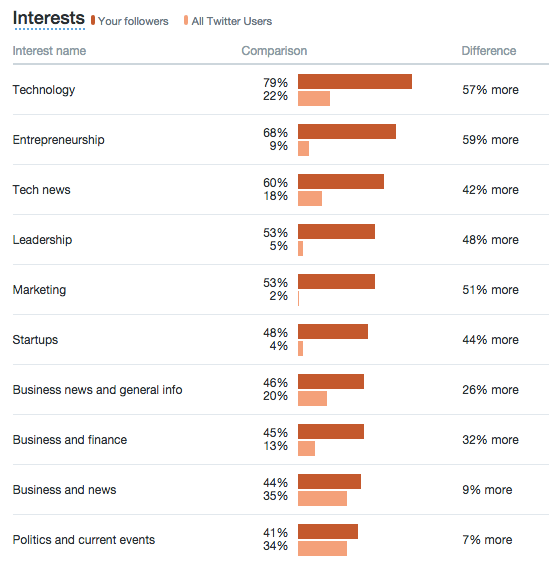
What Drives Growth, Twitter or the Individualized Service?
Twitter is an open social network, meaning that most of the social interactions are visible even to those that are not participating directly. This makes Twitter an inherently viral medium: the more you use it, the greater your growth in followers.
It’s important to understand what aspect of the growth reported above is due to the attention to individuals, as opposed to growth that is attributable to social activity on Twitter.
How We Evaluated the Growth Drivers
Throughout the experiment, we varied the ant’s tweeting activity to assess the impact on growth. The ant’s activities are triggered by follower activity. The ant is alerted to this activity through Twitter’s Streaming API (which is analogous in function to Twitter’s home timeline, as the stream of tweets from accounts you have chosen to follow).
We can vary the amount of activity from the ant from complete inactivity (zero tweets per day) to the maximum quota of tweets provided by Twitter’s public API (2400 tweets per day).
Throughout the beta testing,we also experimented with our use of hashtags, at times excluding hashtags entirely or including up to two hashtags. Our ant is also able to evaluate whether a hashtag is using a broad term or a narrow term (for example, #business compared with #FedRate).
We varied the quality of the hashtags to evaluate the impact within very popular or more niche communities of interest.
What We Learned About Tweet Activity and Hashtags
Our experiments confirmed the obvious, that activity on Twitter drives demand, and that rates of growth in followers can be increased with more frequent tweets. But the details are far more intriguing.
Recall that Primal is using replies to target each tweet to a specific recipient. The use of hashtags extends the reach of the tweet to people that are not following Primal.
When the hashtags we were using were very broad (e.g. #business or #marketing), we lost our ability to target the tweets to specific interests, even when the tweets combined multiple hashtags. In other words, the use of more than one hashtag seemed to broaden the audience, rather than narrow it.
We were able to correct the issue when we composed very specific, individual hashtags, like #WeightGain or #InstituionalInvestors, and precision of the targeting was preserved.
We also experimented with using no hashtags at all, which gave us better visibility into the “word of mouth” growth drivers, predominantly through follower retweets, likes or replies. The contribution to growth of hashtags to follower-referrals was roughly 2:1.
Our conclusion is that Twitter, as a social medium, has an amplifying impact on the growth of a service for individuals, but it also imposes a limiting constraint.
The interests represented by the social graph (for example, through followers-in-common) can easily become misaligned with the interests represented by the interest graph (your individual interests or those represented by communities of interests under hashtags).
This tension between a service organized around individual interests, and Twitter, the social medium, was perhaps most apparent in the motivations for cancelling our service, which we’ll discuss next.
What Causes People to Cancel Their Individual Service?
The most common way to express your dissatisfaction with a service on Twitter is to Unfollow. Unfollowing is a powerful signal of dissatisfaction, so we wanted to dig into the reasons why people choose to unfollow Primal.
How We Analyzed Unfollows
To gain some perspective on what triggered the unfollows, we examined the tweets that were sent to people that unfollowed us. As above, we excluded activity that seemed related to automated activity.
(For example, a large proportion of the accounts that unfollow us are soon thereafter suspended by Twitter. Similarly, another large contingent of accounts systematically delete their tweets, which removes the basis we use for evaluating their interests and the performance of our ant.)
Our expectation was that irrelevance would be the primary driver of unfollows: If people felt our recommendations weren’t relevant to their individual interests, they would unfollow us.
What We Learned About Unfollows
What we discovered baffled us. Almost 75% of the unfollows were related to our inactivity, not our activity.
In the large majority of cases, people unfollow us before we’ve ever addressed a single tweet to them!
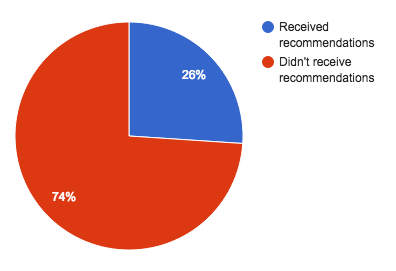
However, this isn’t to say that these unfollowers haven’t seen a tweet from us. Recall that with Twitter’s replies (the special type of tweet that Primal uses), any followers-in-common with both Primal and the target recipient will have an opportunity to see the tweets. If Primal’s tweets are directed to specific recipients, call this secondary audience the indirect recipients of socially recommended tweets.
The high proportion of indirect recipients among the unfollowers is a reflection on whether this social basis for recommending content is effective (or at least congruent with the needs of a service for individuals).
Irrelevance, as discussed in detail below, was implicated in only a small minority of the unfollows among the direct recipients. In contrast, the indirect, social basis was implicated in a majority of the unfollows.
The other important explanation for unfollows speaks to the jobs that people are trying to accomplish through Twitter.
Primal is a personal assistant for content discovery; its job is to provide content that reflects your interests. However, this job may be out of step with many of the other jobs that people are trying to accomplish through Twitter.
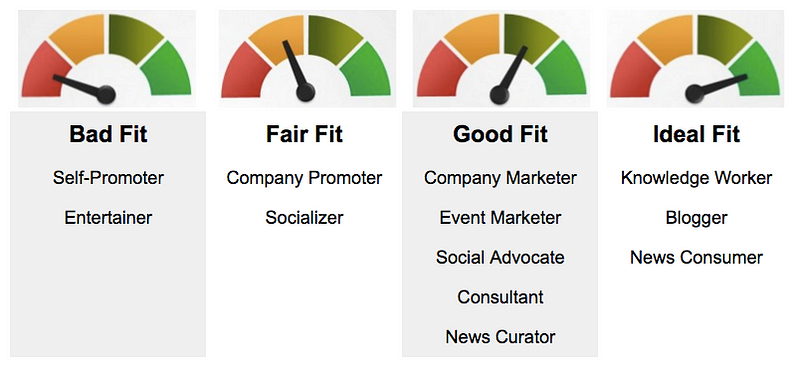
For example, self-promotion is one such job, where the fit with content discovery is questionable. Self-promotion is essentially a selling activity, with little interest in broadening the activity to include related content. Primal’s ant may recommend content that highlights a competing or distracting position, making it an unwelcome participant in the conversation.
Are Individualized Services More Engaging?
Here, user engagement refers to the types and quantities of user interactions with the service. We wanted to investigate whether an individualized service would affect engagement rates, as compared with services that cater to larger groups of people.
How We Compared Engagement Rates
Our expectation was that engagement rates for our individualized service would be higher than the rates reported by companies that cater to larger audiences. We expect that, if the content is targeted precisely to the interests of an individual, it will produce greater engagement than content that is targeted to larger segments of users.
We found it difficult to identify baseline engagement rates, presumably due to concerns within other services about disclosure. We were fortunate, however, to find a thorough report from 2013, from Evolve Capital and Dr. Natalie Petouhoff of the UCLA Anderson School of Management: A Study of The Most Engaged Brand on Twitter: The Most Followed Are Not The Brands With The Highest Engagement. (Comparing numbers reported recently from other sources suggest there’s been very little change in the intervening time.)
Petouhoff ranked 25 brands on Twitter based on follower engagement, as well as reports on the number of followers and tweets-sent. These numbers can be used to compare Primal’s engagement rates against the historical performance of top-performing brands on Twitter.
What We Learned About Engagement for an Individualized Service
Primal’s engagement rates, on a per-follower basis, are more than 5-times higher than what was reported for the top-performing brands. This supports our expectation that individualized services produce higher rates of engagement than services that target their content to larger audiences.
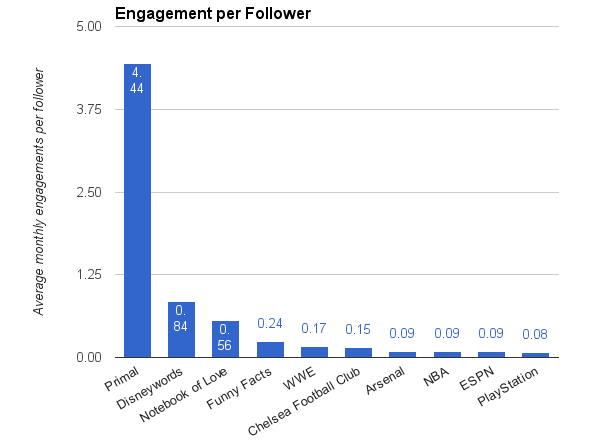
The differences in an individualized service like Primal is most evident in the engagements per tweet. Since each tweet is directed to an audience of one, we would expect the engagements per tweet to reflect this focus. Primal’s average engagements per tweet is 1, where services that are targeted to larger audiences generate much higher engagements for each tweet.
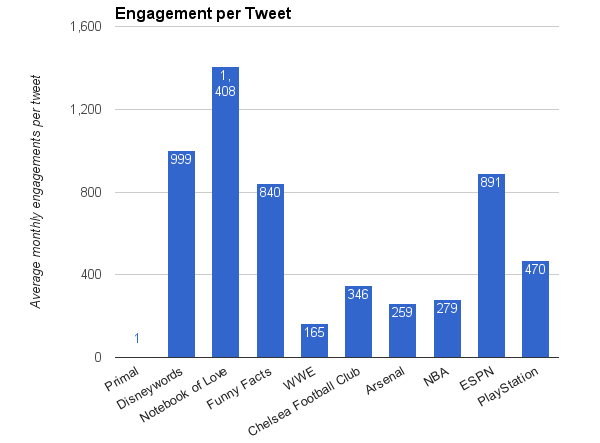
How Does an Individualized Service Make People Feel?
Quantitative engagement metrics alone don’t shed a lot of light on whypeople use a service. To evaluate these questions, we need to dig into the behaviours and sentiments expressed by users through their comments and feedback.
How We Measured User Sentiment
Twitter provides a wealth of information on user sentiments, in the comments and replies to our tweets. While user sentiment is more specific to Primal’s performance as a service, there are a number of conclusions that may be extended to all individualized services.
Positive Sentiments
Overall, the sentiments expressed in response to our service have been very positive, accounting for over 95% of the comments. (Any comments that were addressed to multiple accounts or appeared auto-generated were omitted from the following analysis.)
The tag cloud below represents a textual analysis of comments. The size of the terms indicates their prevalence in the comments, which in turn suggests key themes.
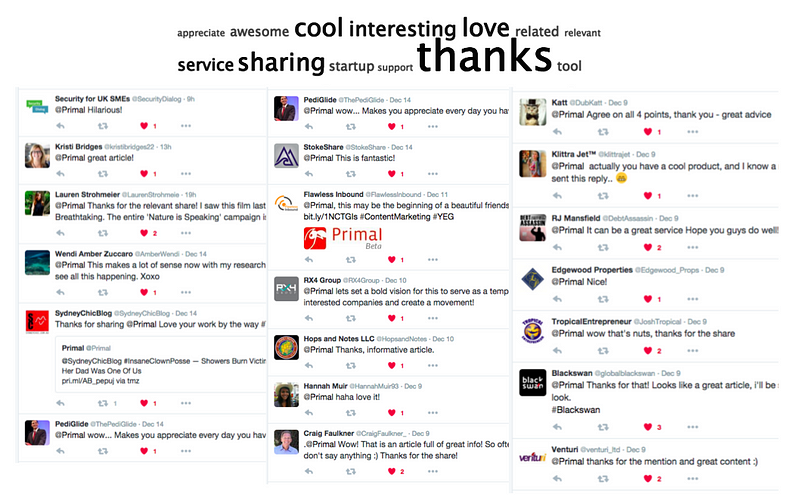
Thanks (for sharing)/appreciate/support was the most common sentiment. It’s a common sentiment for services extended to individuals. We expect that people are much less likely to thank someone if the work product was directed to a large group, rather than to them as an individual. Further, we expect this sentiment to dampen as people realize that a machine is powering the service. (We’ll revisit this question at a later date.)
Awesome/cool/very interesting/love was a slightly less common sentiment than appreciation, but of higher emotional value. Individualized services are uncommon, so there may be a wow-factor to having a significant amount of work product directed to an audience of one. (Frequently, these comments are in response to the individualized pages of content published on Primal.com, which may be perceived as having more work invested in them.)
Perhaps the most surprising aspect of this feedback was how infrequentthe comments were about Primal as a machine-powered service. We expected more feedback about Primal as a tool, but found the comments more directed to Primal as a group of people. (Again, this question will have to be reevaluated once it becomes generally understood that Primal is a machine-powered service.)
Negative Sentiments and Irrelevance
When Primal produces negative sentiment, invariably it’s because the person doesn’t see the relevance of the content recommendation to their interests.
There are a number of graduations of irrelevance. Sometimes, the machine fails to properly interpret the signals of interest from the user’s tweet. For example, a person might tweet about “secrets from an SEO agency”, and Primal concludes that they may be interested in “spies”, working within secret agencies! This is objectively irrelevant.
Content items are rather complex, however, in that they often combine many different themes and ideas. The machine may fail to properly identify the dominant theme or subject within a content item. For example, a content item about, “The Science of Golfing” will have a relative mix of science and golfing subject matter, and that mix can weigh heavily on whether an individual perceives the article as relevant.
Other times, recommended content items are dismissed based on surface level appearances in the titles. The content item might be highly relevant to their interest, but a non-descriptive title fails to communicate the subject matter effectively. (As has been discussed extensively elsewhere, people rarely click through to actually read the content item.)
People hold a higher standard of subjective relevance. The content recommendation must be interesting to me.
More often, however, people hold a higher standard of subjective relevance. That is, the content recommendation must be interesting to me. For example, Primal may conclude that a story about spirituality is relevant to an interest in religion, but people may disagree strenuously about the details of that subject matter. Objectively, it’s relevant, but it may be powerfully disinteresting to the individual involved!
Individualized Services are a Reflection of the Individual
Perhaps the most surprising aspect of negative user sentiment is what it suggests about the emotional weight that individualized services can bring to bear. When Primal misses the mark, it isn’t perceived as merely an irrelevant content recommendation, it’s a misrepresentation of the person, a much more egregious offense.
To put this point in perspective, consider how often your social network contributes content you feel is irrelevant to your individual interests. You might scroll through dozens of items in your social streams before finding one that really reflects your individual interests. In the extreme, it may annoy you, but it probably doesn’t offend you personally.
Individualized services like Primal represent a very different social contract. We’re promising content for you. Consequently, people perceive irrelevance from an individualized service as self-reflection or worse, self-evaluation.
Aparna Chennapragada, head of Google Now, says:
Here, the product is a very high-promise product. What I call the ratio of wow-to-WTF is much (sic) has to be much higher. What I mean by that is that if you get the wrong answer [when] you search for Donald Trump — you get the second-best article, it’s not a big deal right? But if we told you ‘hey, your flight is delayed, don’t worry about it,’ and the goddamn thing is on time … WTF, right?…The stakes are way higher.
Chennapragada is speaking of an aspect that’s general to all software agents, that the degree of autonomy in the software raises the expectations for its performance. But here, we’re arguing that the challenge to personal intelligent assistants is deeper still: the software needs to perform in a manner that expresses the individuality of each end-user.
How Individual Are Twitter Users?
Another presumption is that people on Twitter act like individuals (i.e. they each express very individual interests). Again, while obviously we’re all individuals, do we act that way on a social network? If common interests bind us on a social network, do we express individual interests?
How We Evaluated Individuality
To test the individuality of Twitter users, we generated interest graphs for each follower and then compared the recommendations produced for each. Our expectation was that, if people were expressing themselves in highly individual ways, we would see very little overlap in their content recommendations. On the other hand, if people are tweeting about substantially similar subject matter, we would expect to see considerable overlap in the content recommendations.
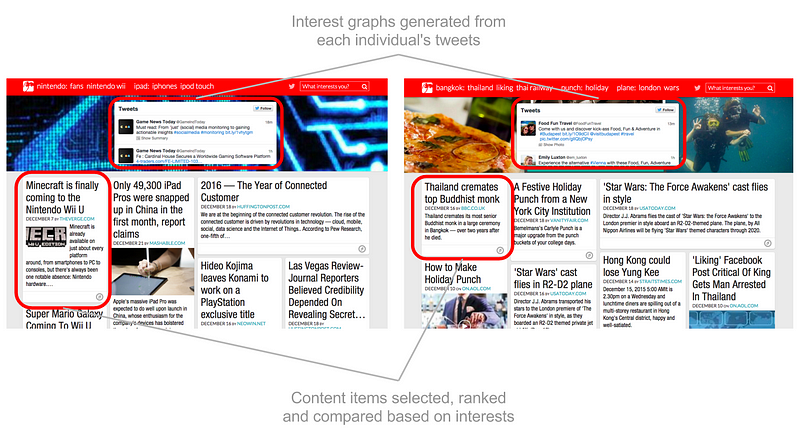
What We Learned About Individuality on Twitter?
People on Twitter behave like individuals. Rarely, a single content item will appeal to a broad audience. However, much more frequently, there is a long tail of content items that align uniquely with individuals.
This individuality is also apparent in the scores that evaluate the degree of interest for each content item. The scores vary widely, with some individuals expressing substantially similar interests and others much more distantly related.
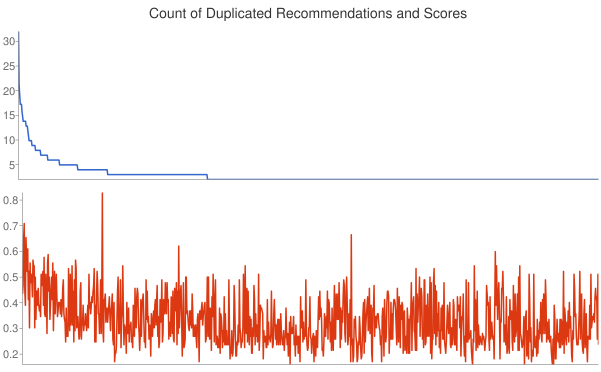
If we zoom in on one particularly interesting article, selected as relevant to many users, this wide distribution in interest becomes readily apparent.
Not only are we all unique individuals, we seem to act that way on Twitter as well.
Part 3: Can Twitter Support Individualized Services?
If you’ve read this far, I hope you’ll agree: the answer to the question of whether Twitter can support services that cater to individuals is a resounding, Yes!
People on Twitter act like individuals; they demand to be treated like individuals. And they reward individualized services with uncommonly high rates of growth, user engagement, and goodwill.
But there are also some considerable challenges, which we believe are systemic to the nature of Twitter as a compound social and interest network.
What follows is a summary of the challenges we encountered in trying to operate a service for individuals on Twitter’s very social network.
The Productivity of a Service for Individuals
In order for an individualized service like Primal to reach its total audience, it has to be much busier than services that cater to larger audiences. If the target audience for each tweet is legitimately one, then you need to send as many tweets as the overall size of your audience.
Technically, this is why services that aspire to individualization require automation to scale to large numbers of users.
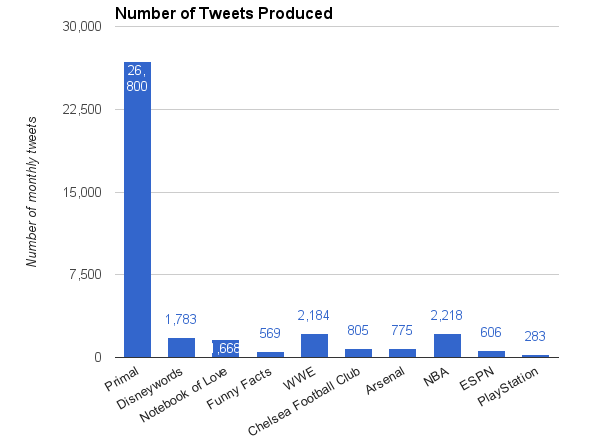
In one-to-one media such as messaging platforms, this productivity isn’t a problem, as users are sheltered from most of the chatter. In a many-to-many medium, such as Twitter, this productivity is a huge challenge. Twitter places strict constraints on the number of tweets that can be sent programmatically across their network. Consequently, the number of people that can be provided this level of individual attention is constrained.
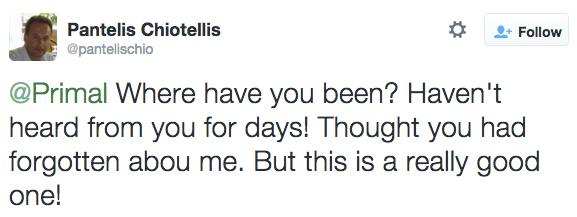
As the service grows, a decision must be made: Do you maintain the segmentation to an audience of one, decreasing the frequency of service, or expand the segments to larger groups, effectively abandoning the individualized nature of the service?
Targeting Based on the Social Graph
Whereas most social services within Twitter would try to maximize the impressions for their tweets, the individualized service needs to precisely target and limit the impressions of its tweets.
Within the social graph, Twitter seems to maintain a strict rein on impressions. We saw no evidence that our tweets extended beyond the boundaries of followers-in-common.
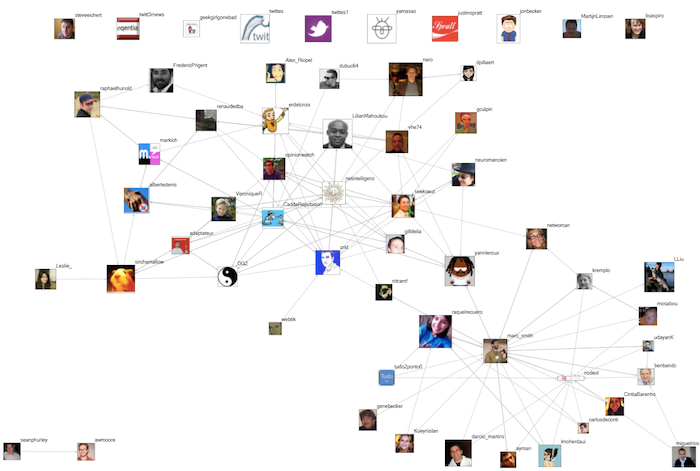
Do followers share the same interests?
The challenge, however, is inherent in the underlying assumption that followers share the same interests. We have direct evidence, in the interest graphs generated for each follower, and indirect evidence in the relatively high rate of unfollows associated with socially recommended content, that followers do not share the same interests, at least not to the level of specificity needed to effectively target content on an individual basis.
Another challenge is the shelf life for tweets. Twitter continues to socialize on your behalf long after you’ve stopped. It isn’t entirely clear where those tweets travel over time. When coupled with the productivity of an individualized service, this represents a significant operational risk.
Targeting Based on the Interest Graph
The greatest challenge in targeting, however, is unambiguously directed to Twitter’s interest graph.
On the surface of it, hashtags are an ideal vehicle for creating communities of interest. However, the underlying mechanism for how to use them is opaque. For example, as is the convention in search, you would expect the use of multiple hashtags to narrow the audience, but on Twitter it appears to do the opposite.
What’s also unclear is the underlying resolution of the Twitter interest graph. To understand this point, consider the resolution of their social graph. Each Twitter account represents a node in the social graph. This resolution is intuitive, transparent and fine-grained, which in turn makes it predictable: If I follow one account, I’ll be socializing with one account.
With Twitter’s interest graph, however, the resolution is fuzzy. What is a node within Twitter’s interest graph: a tweet, a hashtag, a keyword, or combinations thereof? Without this transparency, it becomes difficult to precisely target an audience based on their interests.
Twitter provides interest targeting as a feature of their advertising service: “Interest targeting connects you to a large group of users by prompting you to choose from 25 interest categories that expand into 350 sub-topics…”.
However, in the context of a service for individuals or any service pursuing a sufficiently narrow niche marketing strategy, 350 sub-topics is far too chunky. For example, consider one of these segments, technology. Primal’s relatively small number of followers express a vast range of interests related to technology, summarized in the tag cloud below. As a marketer, do you care whether your technology audience has an interest in security, analytics, or innovation? These are differences that matter.
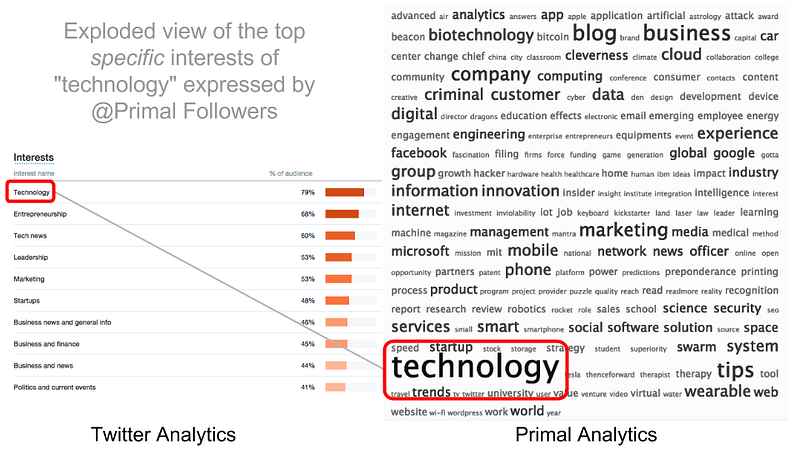
In order to realize Twitter’s true potential as a pure interest network, independent of Twitter the social network, the units of interests must be made far more fine-grained and transparent, and the expression of those interests in the user interfaces predictable.
Twitter’s social graph is sufficient for delivering an individualized service like Primal only because we leverage our own interest graphs. Service-providers need to bring their own data to the table, generated by a community of curators or intelligent machines.
Analytics and Metrics
Usage data is the lifeblood of new product development. Twitter Analytics is invaluable in this respect, providing a generous amount of data to evaluate the performance of your service, as well as an upgrade path to enterprise-grade analytics via Gnip.
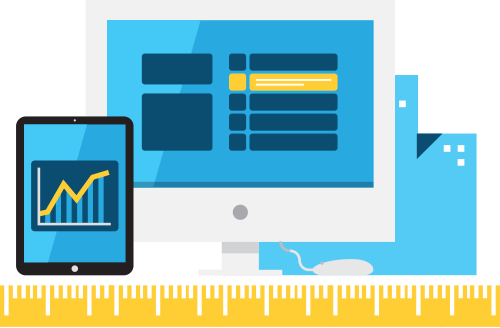
That said, the question here is whether Twitter provides a suitable environment for innovation, specifically, innovation in services targeting individuals. Within that narrow frame, there are two issues worth highlighting: the granularity of the metrics and the need for “negative” metrics.
Twitter, as a social network, provides metrics biased to the values of social services. For example, impressions are considered a key value driver in social services, so Twitter reports performance primarily in terms of impressions. The engagement rate reported by Twitter is the number of engagements divided by the total number of impressions. However, as discussed above, impressions can be a tremendously misleading indicator in the context of a service that targets individuals.
Innovators require very fine-grained metrics that can be used to evaluate new product values. For example, metrics within Twitter such as replies, retweets, and likes are a fantastic start. However, against the backdrop of anonymous “impressions”, it’s difficult to evaluate the actual performance with respect to your target audience.
Even a modest change to segment followers in the analytics would provide tremendous value to individualized services.
The most serious shortcoming in Twitter’s public analytics, however, is the lack of “negative” metrics, or signals that your service is not performing well. Twitter provides user interfaces for expressing negativity: spam reports, blocking users, etc. This information is more vital to the success of an innovative service than the positive metrics. However, negative metrics are not included in Twitter Analytics.
The Future of Twitter: De-Smooshing the Social and Interest Networks
The criticisms levelled at Twitter share a common diagnosis: Tensions arise when different values and organizing bases for information collide within the same user experience.
Twitter is a compound network, one built on the social graph, one on the interest graph. The experience of Twitter is smooshed between the two. It is often unclear how users control the distribution of information across each network, and the algorithmic bases for Twitter’s targeting are even more opaque.
Throughout this report, the challenges to effectively targeting information to individuals distill to this same tension: We want to target information based on individual interests, but we need to piggyback on Twitter’s social graph to pursue it.
Decoupling the interest network from the social network would alleviate tensions that underlie most criticisms of Twitter, as well as catalyze tremendous growth opportunities.
The resolution of this tension has two aspects: a) introducing a mechanism for user-directed interest networking; and b) algorithmic targeting that decouples the social and interest networks.
User-Directed Interest Networking
Twitter already has a simple convention for user-directed targeting of information within the social graph. Replies are targeted to narrower audiences by beginning a tweet with the target recipient.
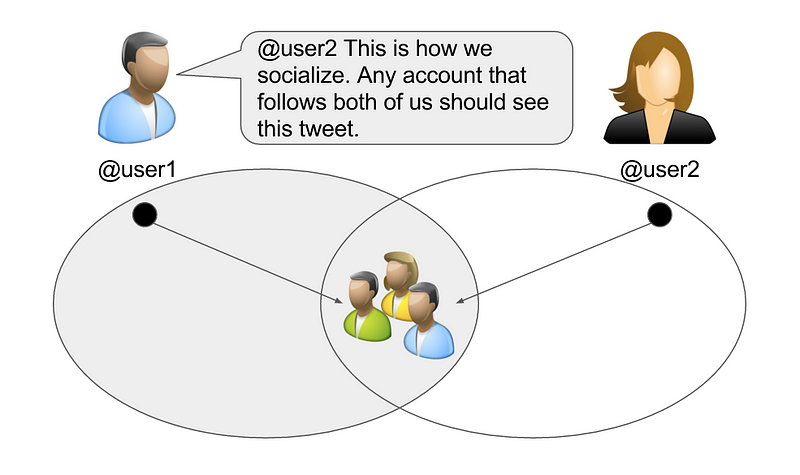
A similar opportunity is available with their interest network, where a specific area of interest, identified by a hashtag, leads the tweet.
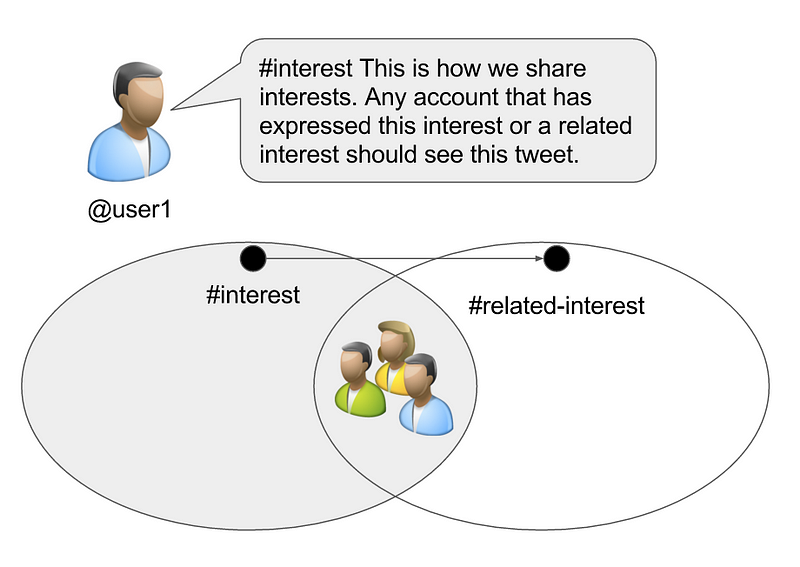
If people wanted to target a tweet using interests (hashtags) and highlight the information within their social network (mentions), the two bases could still be combined within a single tweet.
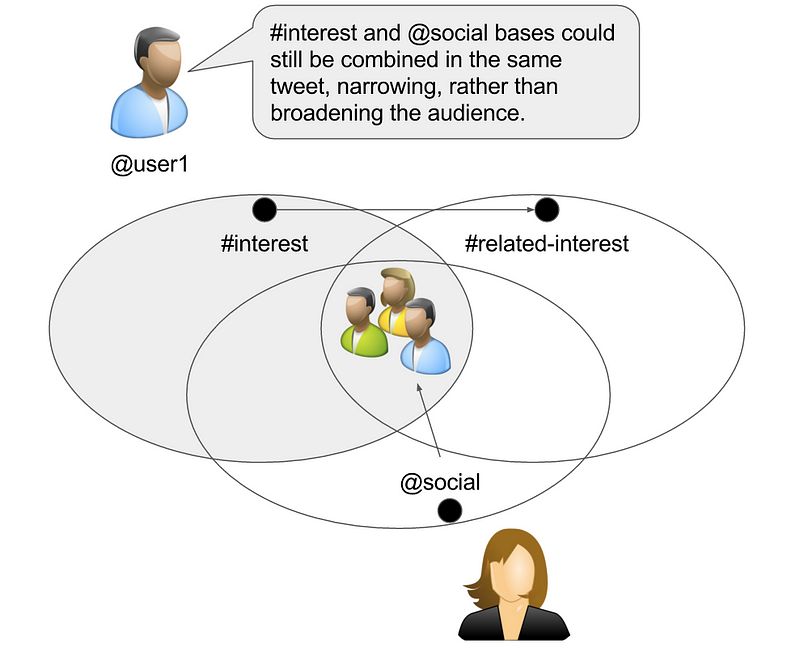
The difference between what’s envisioned here and how Twitter works now is in a decoupling of the social and interest-based experiences.
Presently, if you begin your tweet with a hashtag it will be broadcast to all your followers, regardless of whether they share that interest. The hashtag broadens the reach of your tweet in a fairly indiscriminate and self-defeating way.
Instead, adding additional hashtags and mentions should narrow the audience. If you include more than one hashtag or mention in your tweet, your tweet should become relevant to a smaller audience, not larger.
Interest-based Algorithmic Targeting
The vast majority of the Twitter experience is mediated by machines, not people. Algorithms determine which of the many potentially relevant tweets you actually get to see.
Therefore, the de-smooshing of the Twitter experience needs to extend down to the algorithmic level. User-directed targeting, discussed above, and algorithmic targeting must be congruent, transparent and predictable.
Ultimately, success on this front demands far more fine-grained interest graphs.
As an organizing basis, interests needs to be at least as discriminating as the social graph (that is, supporting small groups as well as large groups). In fact, based on our testing, an appropriate level of resolution is several orders of magnitude greater than the social graph.
The actual resolution that can be cost-effectively supported by Twitter is a question well beyond our visibility, but we can make a few generalizations based on our experience, reported herein:
- Twitter’s interest graph should reflect the individuality of Twitter users. Solutions that collate large numbers of users within relatively broad categories of interest will fail to provide a sufficient degree of resolution for effective targeting. Primal’s interest graphs are generated for every user, with a resolution down to the individual tweets being expressed. We’ve also demonstrated effective targeting based on Twitter keyword searches and individual hashtags.
- Semantic descriptions of interests is a necessary and sufficient basis for targeting to individual interests. While Primal does sort content based on secondary bases such as the authority of the publisher and the timeliness of the content, the semantic relevance between the tweets expressed by each user and the target content is the only matching criterion.
- The costs of generating interest graphs can be constrained, growing linearly with the number of users as opposed to exponentially with the number of distinct interests expressed. In other words, without knowing anything about Twitter’s cost structure for generating interest graphs, we can conclude that interest graphs extended to Twitter’s scale is economically viable.
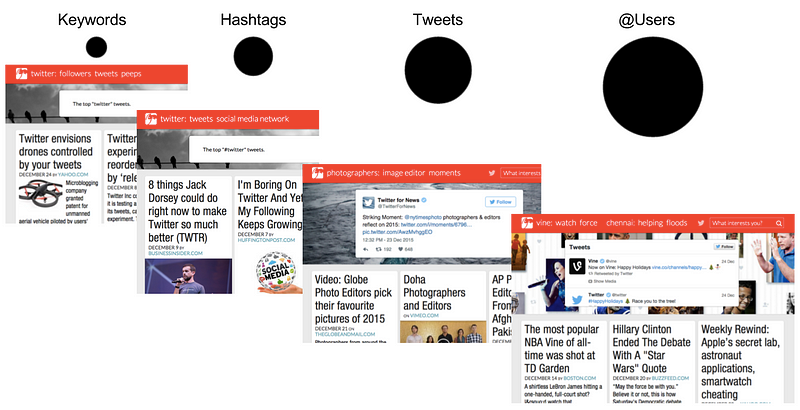
Benefits to End-Users
What are the benefits of a de-smooshed Twitter? Chris Sacca offers a useful frame on the problems:
- For most people, Twitter is too hard to use.
- For most people, Tweeting is scary.
- For most people, Twitter feels lonely.
Here, we argue that all these high-level problems follow from the conflation of Twitter the social network with Twitter the interest network.
Twitter is hard to use because you need to curate a social network to follow your interests. For new users, this burden represents a barrier to getting started. For existing users, it represents an ongoing chore that isn’t sociable or interesting. This coupling is not only time-consuming, it doesn’t work very well, since we each hold and express very individual interests.
This coupling between the social and interest networks is scary because it forces people to socialize when they don’t want to. Users of an interest network shouldn’t have to socialize to get value. To this problem, many propose tactics that help people socialize, when there’s a simpler solution:
Don’t force behaviours that are tangential to the goal of discovering interesting information.
There’s a similar prescription to the loneliness concern: embrace more individual and personal experiences of Twitter. No one bemoans the loneliness of interesting content. As a fully realized interest network, people would curl up with Twitter like they curl up with a great book. Those on the periphery of Twitter’s social network could each be made the focal point of Twitter’s interest network.
This may all seem anathema to the proponents of Twitter as a social network. To be clear, this embrace of interest networking in no way undermines Twitter’s social networking activities. The benefits run in both directions. A more organized interest network creates a more organized social network.
Business Benefits
The essence of Twitter’s value proposition to marketers and advertisers lies in the precision of their targeting capabilities. How do you match the right content and messaging to the right audience? By investing in their interest graph and differentiating between the social and interest networks, Twitter would not only improve the efficiency of their business solutions but simplify the customer experience, as well.
The inconveniences and inefficiencies that burden end-users burden customers as well. It’s painful to describe a target of interest using bases that describe social networks.
Perhaps most important to their stakeholders, the interest network represents Twitter’s greatest growth opportunity. It’s difficult to fathom, but Twitter’s latent interest graph is many orders of magnitude larger than its social graph. The breadth of interest-based services and experiences that it could support would dwarf the scope of social networks.
Twitter’s greatest future is the first, fully realized, mass market interest network.
Acknowledgements
Our Followers: First and foremost, we want to thank the many people on Twitter that have participated directly in our beta, through their use of the service and their feedback. Test-driving a legitimately new type of service doesn’t come without it’s fair share of WTFs, fails and missteps. We sincerely appreciate your patience and participation.
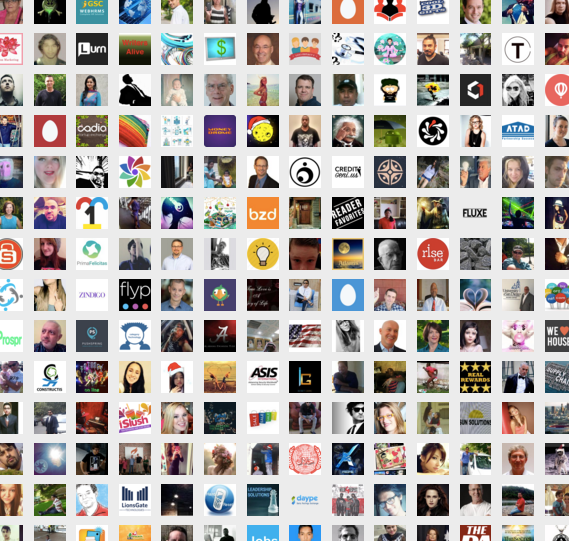
Twitter: Primal’s individualized service is unique, and uniqueness raises all sorts of alarms within a complex network like Twitter. The team at Twitter could have easily evicted Primal as a service that doesn’t fit the mold, but they didn’t do that. They worked with us to help identify issues, provide feedback, and refine the service.
Our team: Small, smart teams can tackle really big problems. If you believe that searching for information is a problem, that the right information should just find you, we’d love to hear from you!
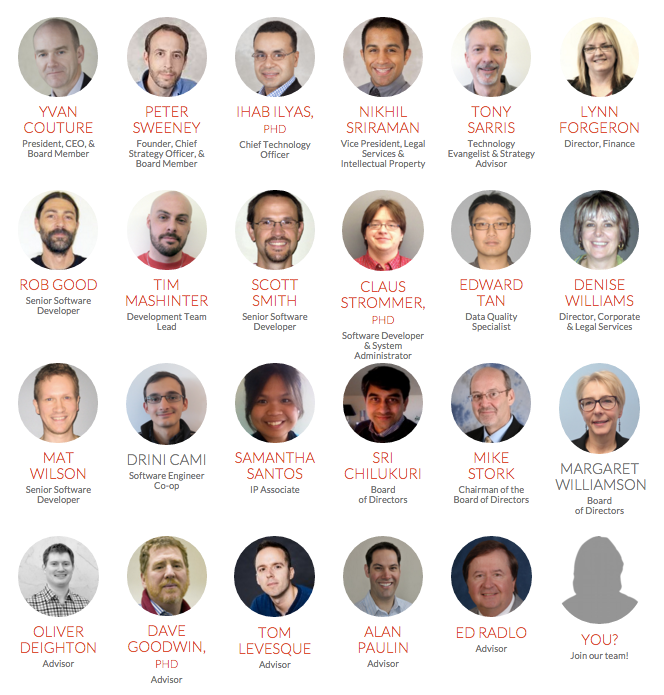