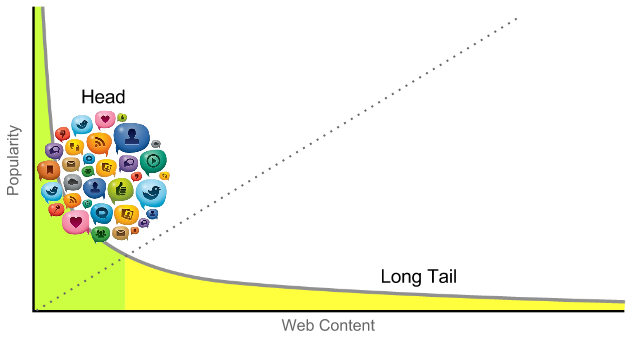
What Amazon teaches us about the long tail market for content discovery
The market for content discovery is largely unclaimed. Incumbents are focusing on content discovery for the masses, neglecting the long tail of recommendations for individuals.
The barrier to this long tail market is contextual knowledge, specific to you and your interests at this moment in time.
Context is a pretty abstract concept, so let’s explore some examples to make things more concrete.
A recommender field study in the Amazon…
Amazon is generally regarded as the king of the recommendations mountain.
Here, the domain of knowledge is books; the context for each request is the specific book that the consumer is browsing. To make intelligent recommendations, Amazon needs knowledge of both the domain and the context.
The first example is a New York times best-seller.
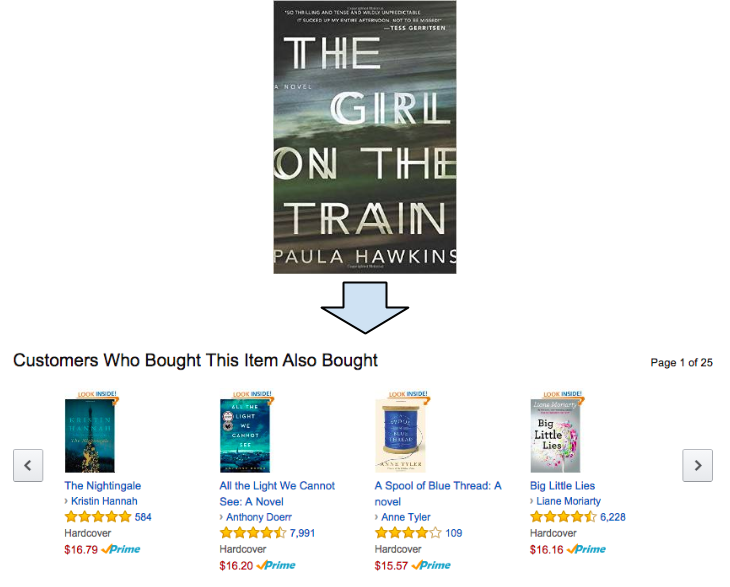
This example, as a popular best-seller, derives its recommendations from a wealth of information, including domain-specific knowledge (such as lists of best-sellers) and its popularity (the ample browsing behaviours of people that are interested in this book).
The context of the best-selling book is a good match for Amazon’s knowledge, and the recommendations seem intelligent for it.
The second example is one of the worst-reviewed books on Amazon.
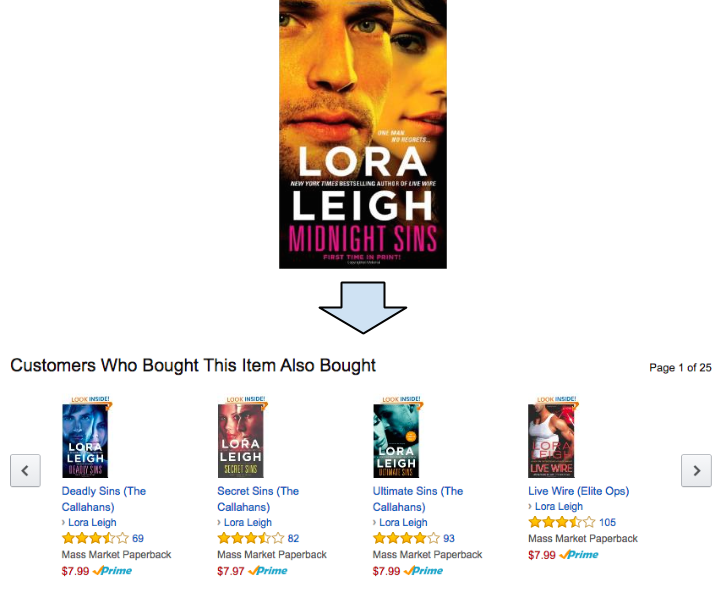
In this counterexample, the knowledge representations are comparatively modest. It’s hard to find lists of unpopular books and the inventory of browsing behaviours for unpopular books are typically sparse.
We’re left with recommendations that reflect the limited knowledge Amazon has about the book (such as the author, Lora Leigh). Consequently, the recommendations — books by this author — seem far less intelligent for these gaps in Amazon’s knowledge.
The long tail of recommendations
The examples above characterize a long tail problem.
With the popular book, there is ample knowledge within Amazon to reflect the context of my interests; with the unpopular book, the knowledge of my interests thins out considerably.
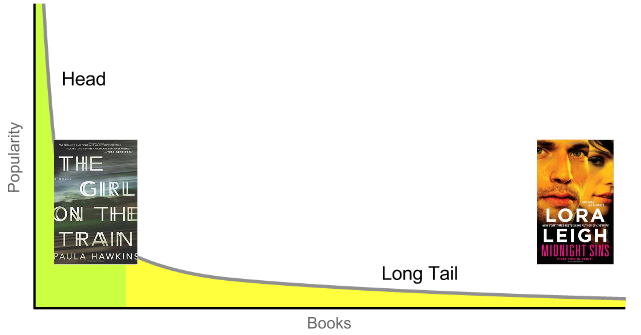
Back to the premise: In order to make good recommendations, the recommendation system not only needs general-purpose domain knowledge, but also knowledge that’s specific to the context for the request. Recommenders typically fail in the long tail where this contextual knowledge is sparse.
The frontier of content discovery
Who cares about unpopular books? Well, individuals. And in the aggregate, large numbers of individuals account for very large markets. (Over a third of Amazon’s sales are niche books!)
Amazon is a microcosm of the web. The performance of today’s recommenders reflects this bias towards popular, head-of-the-curve, content.
The unclaimed frontier of content discovery is in the long tail of our individual and highly contextual interests.